In this course you learn to build, refine, extrapolate, and, in some cases, interpret models designed for a single, sequential series. There are three modeling approaches presented. The traditional, Box-Jenkins approach for modeling time series is covered in the first part of the course. This presentation moves students from models for stationary data, or ARMA, to models for trend and seasonality, ARIMA, and concludes with information about specifying transfer function components in an ARIMAX, or time series regression, model. A Bayesian approach to modeling time series is considered next. The basic Bayesian framework is extended to accommodate autoregressive variation in the data as well as dynamic input variable effects. Machine learning algorithms for time series is the third approach. Gradient boosting and recurrent neural network algorithms are particularly well suited for accommodating nonlinear relationships in the data. Examples are provided to build intuition on the effective use of these algorithms.

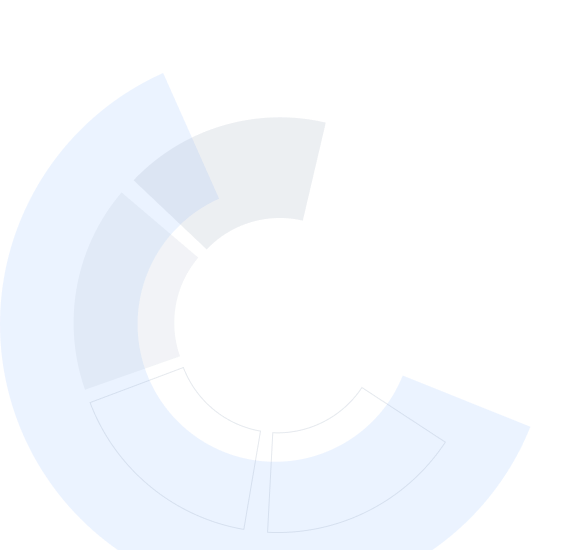
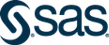
Modeling Time Series and Sequential Data
This course is part of Analyzing Time Series and Sequential Data Specialization



Instructors: Chip Wells
Included with
Details to know

Add to your LinkedIn profile
19 assignments
See how employees at top companies are mastering in-demand skills
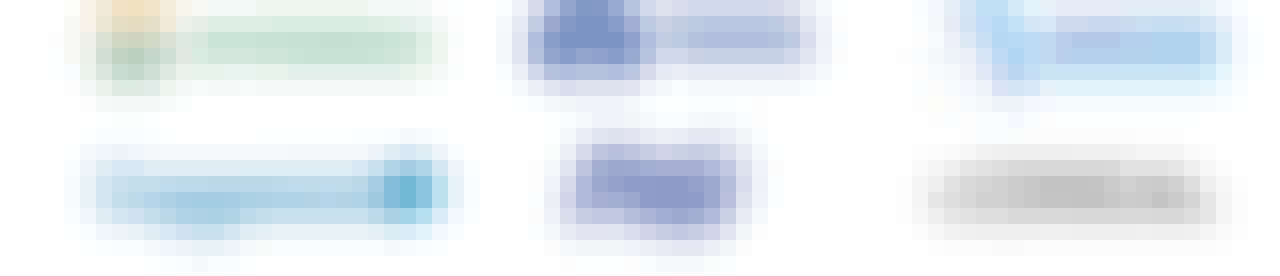
Build your subject-matter expertise
- Learn new concepts from industry experts
- Gain a foundational understanding of a subject or tool
- Develop job-relevant skills with hands-on projects
- Earn a shareable career certificate
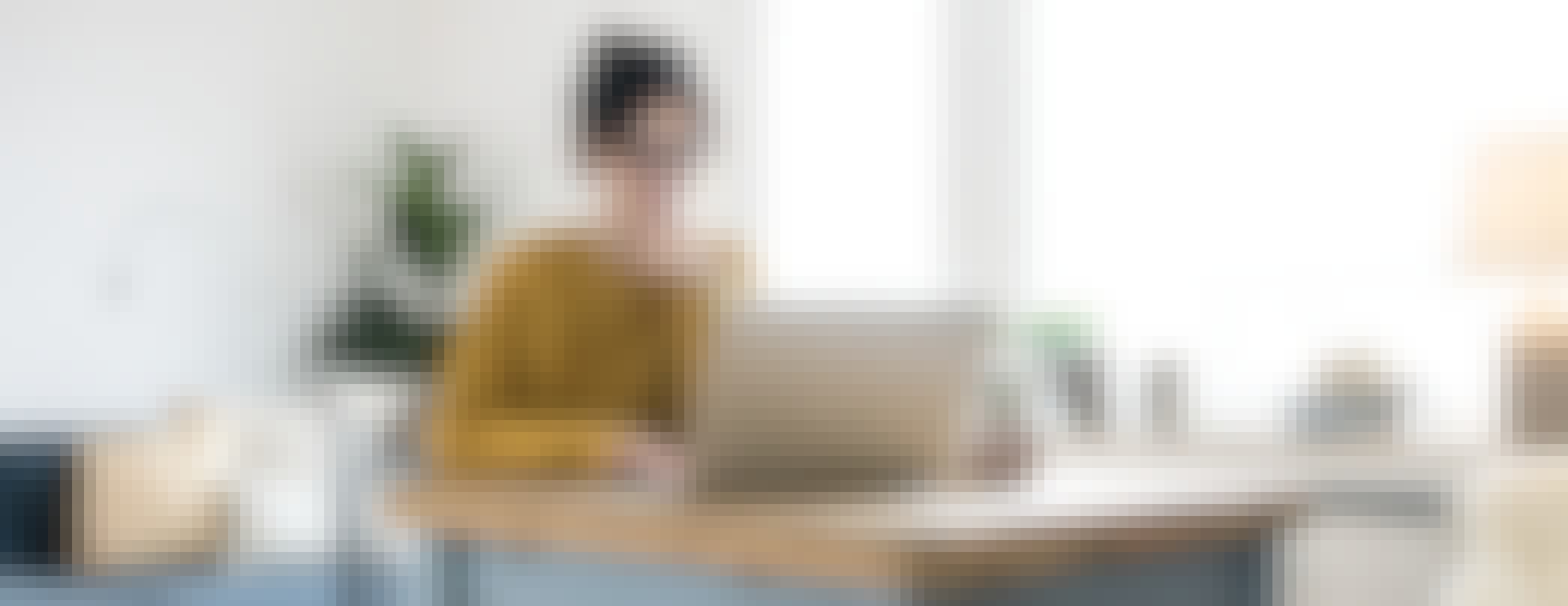
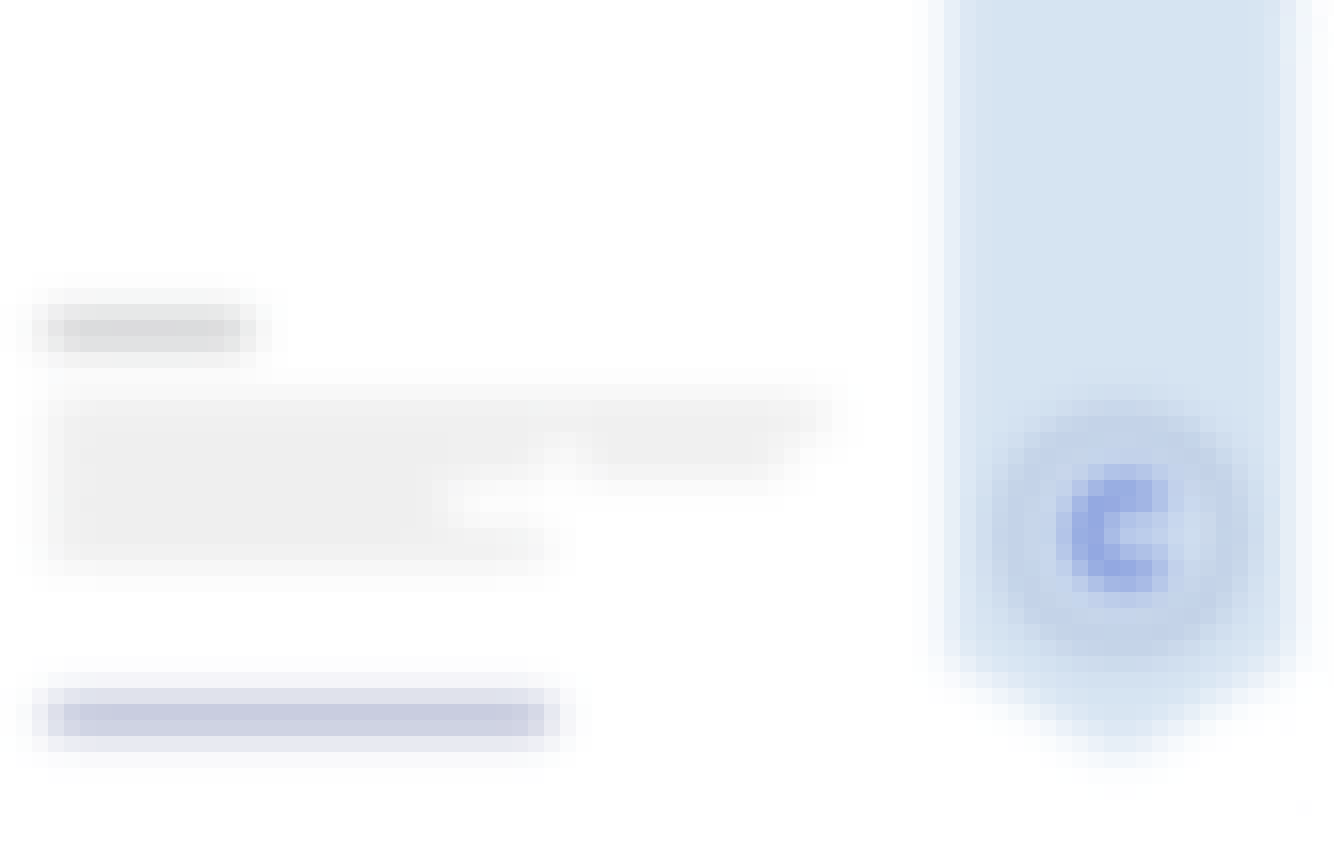
Earn a career certificate
Add this credential to your LinkedIn profile, resume, or CV
Share it on social media and in your performance review
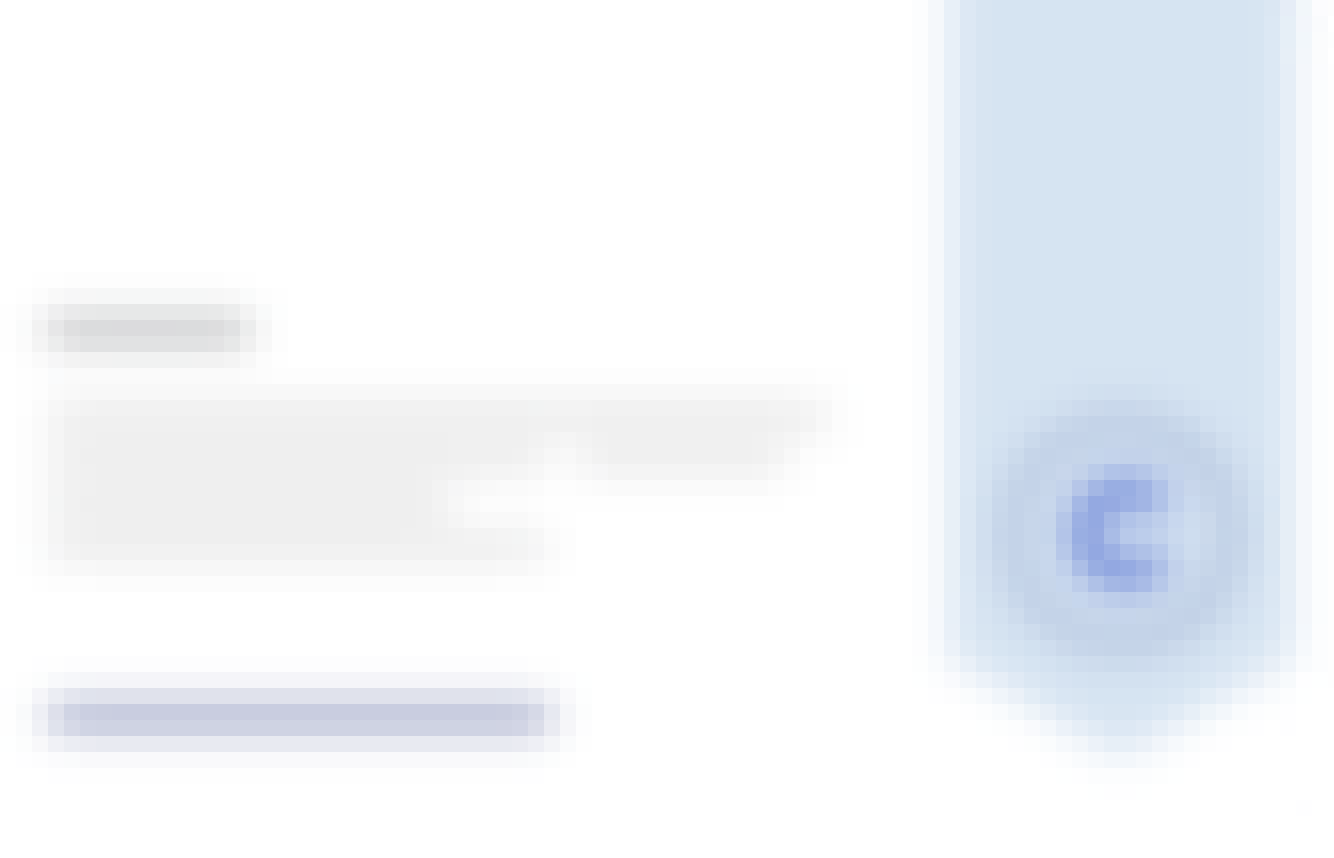
There are 8 modules in this course
In this module you get an overview of the courses in this specialization and what you can expect.
What's included
1 video1 reading
In this module, you get an idea of the scope of this course and learn to use SAS Viya for Learners to do the practices in the course.
What's included
1 video2 readings1 app item
This module reviews fundamental time series ideas. You learn about the basic components of systematic variation in time series data and some simple model specifications, such as the autoregressive order one and the random walk. You also learn about Exponential smoothing models or ESMs, selecting a champion ESM, and generating forecasts on time series.
What's included
11 videos2 assignments1 app item
This module has four parts. The first part describes traditional models for stationary data: Auto Regressive Moving Average or ARMA models. The second part describes how the ARMA framework is generalized to accommodate trend variation. This involves integration, and results in the ARIMA model. The third part describes how the ARIMA model is adapted to handle seasonal variation in the data. The fourth and final part of the module introduces the dynamic regression or ARIMAX model and describes concepts related to identifying transfer function components and specifying ARIMAX models.
What's included
26 videos2 assignments1 app item
In this module, we combine the worlds of time series and Bayesian analysis. We begin with a brief review of Bayesian analysis. We then explore how to incorporate autoregressive, seasonal, and exogenous components in a Bayesian time series. We conclude with a discussion on Bayesian scoring and posterior predictive distributions.
What's included
10 videos8 assignments1 app item
In this module you learn how to use SAS machine learning tools to forecast individual time series. You learn to prepare the time series data for use with the machine learning tools, and how to build and score forecasting models using these tools. We focus on gradient boosting and recurrent neural network models and discuss when it would be useful to use these methods.
What's included
8 videos1 reading5 assignments1 app item
This module describes how forecasts that are generated externally to the forecasting system can be accommodated in SAS Visual Forecasting. We'll use external forecasts to create a combined or ensemble forecast that has the potential to improve forecast precision relative to the constituent, external forecasts. This module concludes with a discussion of hybrid model forecasts that combine traditional and machine learning approaches to forecasting.
What's included
9 videos1 assignment2 app items
What's included
1 assignment
Instructors



Offered by
Explore more from Data Analysis
Why people choose Coursera for their career




New to Data Analysis? Start here.

Open new doors with Coursera Plus
Unlimited access to 10,000+ world-class courses, hands-on projects, and job-ready certificate programs - all included in your subscription
Advance your career with an online degree
Earn a degree from world-class universities - 100% online
Join over 3,400 global companies that choose Coursera for Business
Upskill your employees to excel in the digital economy
Frequently asked questions
Access to lectures and assignments depends on your type of enrollment. If you take a course in audit mode, you will be able to see most course materials for free. To access graded assignments and to earn a Certificate, you will need to purchase the Certificate experience, during or after your audit. If you don't see the audit option:
The course may not offer an audit option. You can try a Free Trial instead, or apply for Financial Aid.
The course may offer 'Full Course, No Certificate' instead. This option lets you see all course materials, submit required assessments, and get a final grade. This also means that you will not be able to purchase a Certificate experience.
When you enroll in the course, you get access to all of the courses in the Specialization, and you earn a certificate when you complete the work. Your electronic Certificate will be added to your Accomplishments page - from there, you can print your Certificate or add it to your LinkedIn profile. If you only want to read and view the course content, you can audit the course for free.
If you subscribed, you get a 7-day free trial during which you can cancel at no penalty. After that, we don’t give refunds, but you can cancel your subscription at any time. See our full refund policy.
More questions
Financial aid available,