In this 1-hour long project, you will learn how to generate your own high-dimensional dummy dataset. You will then learn how to preprocess it effectively before training a baseline PCA model. You will learn the theory behind the autoencoder, and how to train one in scikit-learn. You will also learn how to extract the encoder portion of it to reduce dimensionality of your input data. In the course of this project, you will also be exposed to some basic clustering strength metrics.

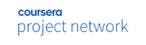
Dimensionality Reduction using an Autoencoder in Python
Taught in English

Instructor: Ari Anastassiou
3,876 already enrolled
Included with
Guided Project
Recommended experience
(99 reviews)
What you'll learn
How to generate and preprocess high-dimensional data
How an autoencoder works, and how to train one in scikit-learn
How to extract the encoder portion from a trained model, and reduce dimensionality of your input data
Skills you'll practice
Details to know

Add to your LinkedIn profile
Guided Project
Recommended experience
(99 reviews)
See how employees at top companies are mastering in-demand skills
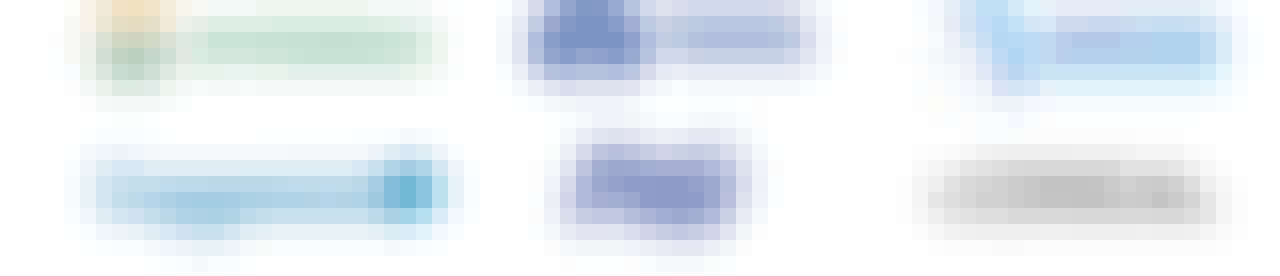
Learn, practice, and apply job-ready skills in less than 2 hours
- Receive training from industry experts
- Gain hands-on experience solving real-world job tasks
- Build confidence using the latest tools and technologies
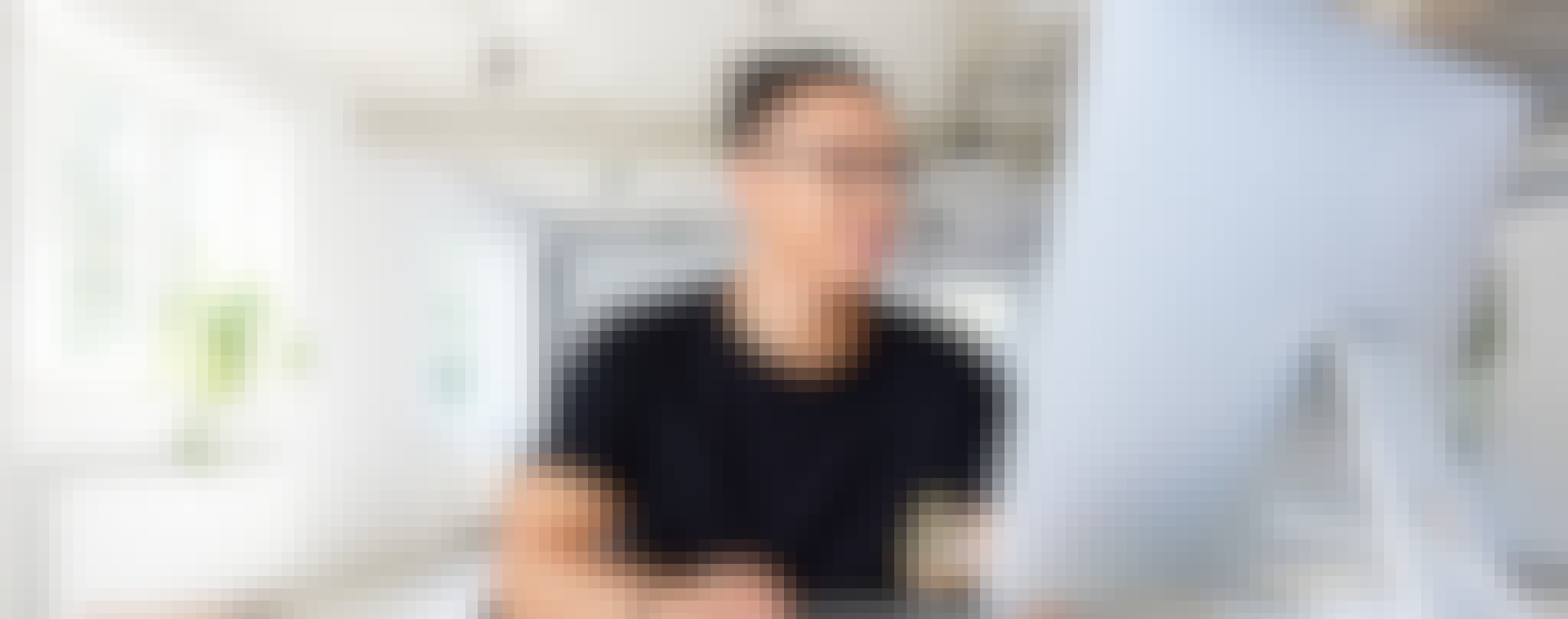
About this Guided Project
Learn step-by-step
In a video that plays in a split-screen with your work area, your instructor will walk you through these steps:
An introduction to the problem and a summary of needed imports
Dataset creation and preprocessing
Using PCA as a baseline for model performance
Theory behind the autoencoder architecture and how to train a model in scikit-learn
Reducing dimensionality using the encoder half of an autoencoder within scikit-learn
Recommended experience
Intermediate Python users with some exposure to neural networks.
3 project images
Instructor

Offered by
How you'll learn
Skill-based, hands-on learning
Practice new skills by completing job-related tasks.
Expert guidance
Follow along with pre-recorded videos from experts using a unique side-by-side interface.
No downloads or installation required
Access the tools and resources you need in a pre-configured cloud workspace.
Available only on desktop
This Guided Project is designed for laptops or desktop computers with a reliable Internet connection, not mobile devices.
Why people choose Coursera for their career




Learner reviews
Showing 3 of 99
99 reviews
- 5 stars
67.67%
- 4 stars
24.24%
- 3 stars
7.07%
- 2 stars
1.01%
- 1 star
0%
New to Machine Learning? Start here.

Open new doors with Coursera Plus
Unlimited access to 7,000+ world-class courses, hands-on projects, and job-ready certificate programs - all included in your subscription
Advance your career with an online degree
Earn a degree from world-class universities - 100% online
Join over 3,400 global companies that choose Coursera for Business
Upskill your employees to excel in the digital economy
Frequently asked questions
By purchasing a Guided Project, you'll get everything you need to complete the Guided Project including access to a cloud desktop workspace through your web browser that contains the files and software you need to get started, plus step-by-step video instruction from a subject matter expert.
Because your workspace contains a cloud desktop that is sized for a laptop or desktop computer, Guided Projects are not available on your mobile device.
Guided Project instructors are subject matter experts who have experience in the skill, tool or domain of their project and are passionate about sharing their knowledge to impact millions of learners around the world.