This course introduces basic time series analysis and forecasting methods. Topics include stationary processes, ARMA models, modeling and forecasting using ARMA models, nonstationary and seasonal time series models, state-space models, and forecasting techniques.

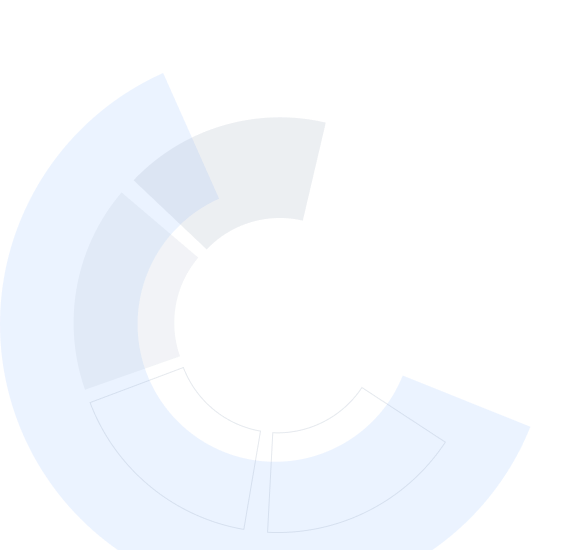
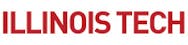
Recommended experience
Skills you'll gain
Details to know

Add to your LinkedIn profile
27 assignments
See how employees at top companies are mastering in-demand skills
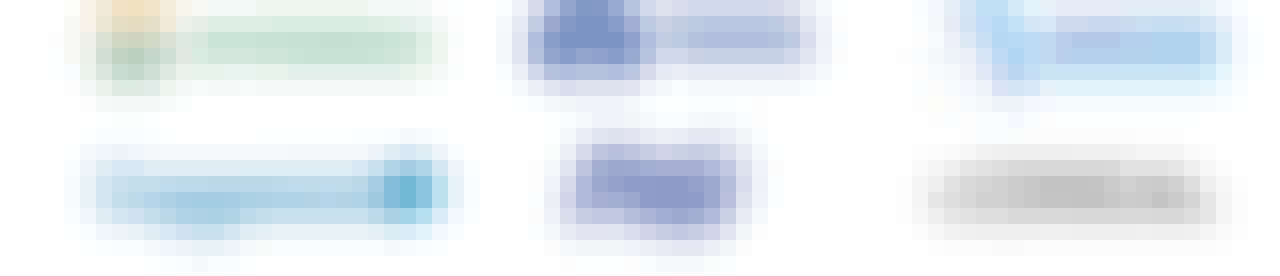
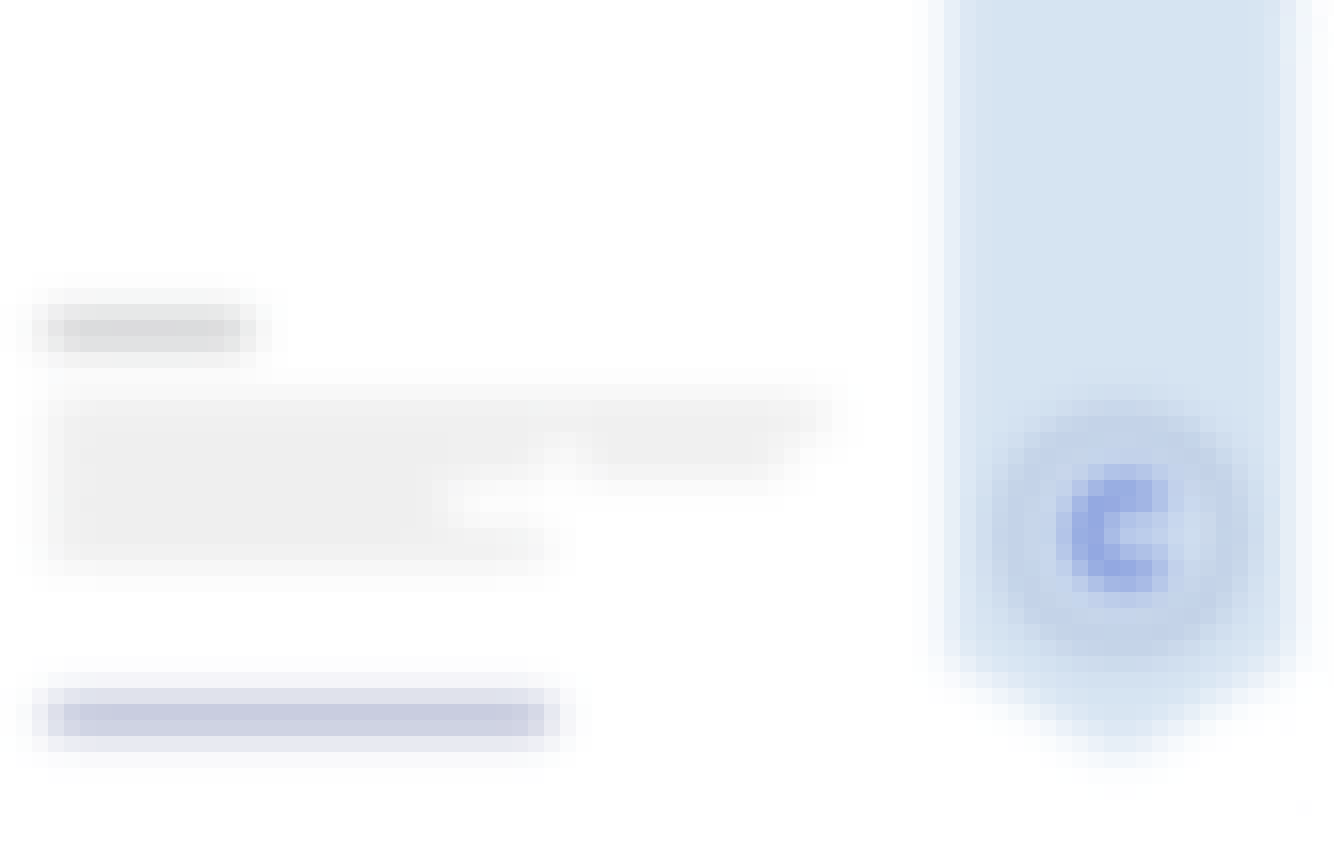
Earn a career certificate
Add this credential to your LinkedIn profile, resume, or CV
Share it on social media and in your performance review
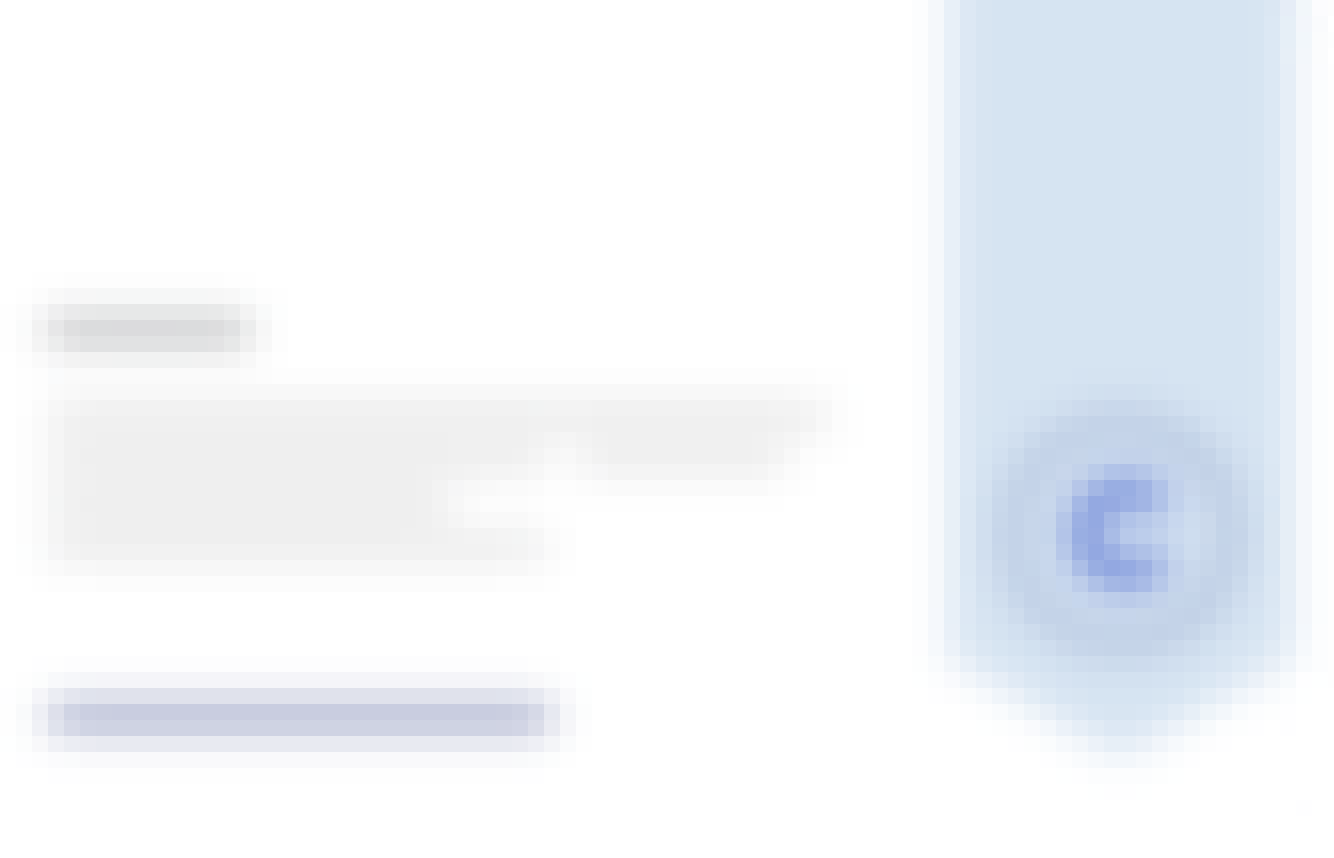
There are 9 modules in this course
Welcome to Introduction to Time Series! In this module we'll define time series and time series models, and we'll develop some intuition for the fundamental concept of stationarity, and why it's useful.
What's included
8 videos5 readings4 assignments1 discussion prompt
In this module, we'll discuss stationarity in more detail. We'll learn the technical definitions of weak and strong stationarity, and explain why the weaker version is more practical to use. We'll discuss the autocovariance and autocorrelation functions for stationary processes---concepts that will be with us for the rest of the course. And finally, we'll see some examples of ARMA processes, which we'll treat more deeply in the coming modules.
What's included
9 videos3 readings3 assignments
In this module, we'll focus on ARMA processes, and what is arguably their most important feature, namely their autocorrelation structure. We'll see how to compute these "from scratch" (with a little help from R for the computations), and look at plots of the autocorrelation function (ACF) to get some intuition for how the ACF of an ARMA process behaves and what it can tell us.
What's included
10 videos4 readings3 assignments
In this module, we begin by discussing the ACF's of more complicated ARMA processes. Our main focus, though, is on one-step-ahead forecasts. We learn about the best linear predictor: both how it is defined and how to use it. Finally, we use what we have learned in order to define the Partial Autocorrelation Function (PACF), which is another fundamental tool in the study of stationary processes.
What's included
9 videos3 readings3 assignments
In this module, we learn about fitting a stationary time series model to data. The fitting process involves determining what values of the parameters to use. We discuss preliminary estimation and maximum likelihood estimation of these parameters.
What's included
9 videos4 readings4 assignments
In this module, we discuss model diagnostics and order selection. Given an ARMA order, we've already seen how to best fit the parameters of the associated model. Given several different fitted models, the tools we develop in this module will allow us to make an intelligent choice about which one to use.
What's included
7 videos3 readings3 assignments
This module introduces students to ARIMA and SARIMA modeling techniques, essential for analyzing non-stationary and seasonal time series data. In the first lesson, students will learn to define ARIMA processes, use the Dickey-Fuller test to determine the need for differencing, and fit ARIMA models using R. The second lesson extends these skills to SARIMA models, focusing on identifying seasonality and fitting these models to capture seasonal patterns in data.
What's included
9 videos3 readings3 assignments
This module equips students with more sophisticated forecasting techniques beyond one-step-ahead predictions. We treat both (S)ARIMA models and exponential smoothing models and show how to handle forecasts in R. For the simplest of these models, we look inside the "black box" a little bit and demonstrate how these forecasts are generated.
What's included
9 videos3 readings3 assignments
This module contains the summative course assessment that has been designed to evaluate your understanding of the course material and assess your ability to apply the knowledge you have acquired throughout the course.
What's included
1 assignment
Instructor

Offered by
Explore more from Data Analysis
Build toward a degree
Why people choose Coursera for their career




New to Data Analysis? Start here.

Open new doors with Coursera Plus
Unlimited access to 10,000+ world-class courses, hands-on projects, and job-ready certificate programs - all included in your subscription
Advance your career with an online degree
Earn a degree from world-class universities - 100% online
Join over 3,400 global companies that choose Coursera for Business
Upskill your employees to excel in the digital economy
Frequently asked questions
Access to lectures and assignments depends on your type of enrollment. If you take a course in audit mode, you will be able to see most course materials for free. To access graded assignments and to earn a Certificate, you will need to purchase the Certificate experience, during or after your audit. If you don't see the audit option:
The course may not offer an audit option. You can try a Free Trial instead, or apply for Financial Aid.
The course may offer 'Full Course, No Certificate' instead. This option lets you see all course materials, submit required assessments, and get a final grade. This also means that you will not be able to purchase a Certificate experience.
When you enroll in the course, you get access to all of the courses in the Specialization, and you earn a certificate when you complete the work. Your electronic Certificate will be added to your Accomplishments page - from there, you can print your Certificate or add it to your LinkedIn profile. If you only want to read and view the course content, you can audit the course for free.
If you subscribed, you get a 7-day free trial during which you can cancel at no penalty. After that, we don’t give refunds, but you can cancel your subscription at any time. See our full refund policy.
More questions
Financial aid available,