In this 2-hour long project-based course, you will learn the basics of using weight regularization and dropout regularization to reduce over-fitting in an image classification problem. By the end of this project, you will have created, trained, and evaluated a Neural Network model that, after the training and regularization, will predict image classes of input examples with similar accuracy for both training and validation sets.

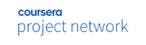
Avoid Overfitting Using Regularization in TensorFlow
Taught in English

Instructor: Amit Yadav
4,512 already enrolled
Included with
Guided Project
Recommended experience
(76 reviews)
What you'll learn
Develop an understanding on how to avoid over-fitting with weight regularization and dropout regularization
Be able to apply both weight regularization and dropout regularization in Keras with TensorFlow backend
Skills you'll practice
Details to know

Add to your LinkedIn profile
Guided Project
Recommended experience
(76 reviews)
See how employees at top companies are mastering in-demand skills
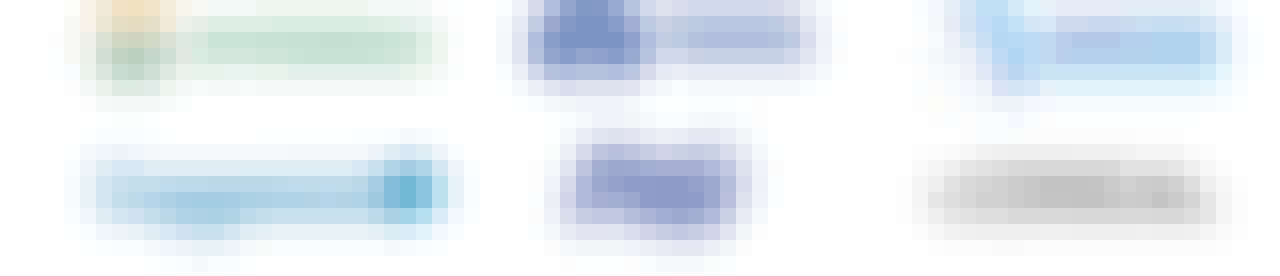
Learn, practice, and apply job-ready skills in less than 2 hours
- Receive training from industry experts
- Gain hands-on experience solving real-world job tasks
- Build confidence using the latest tools and technologies
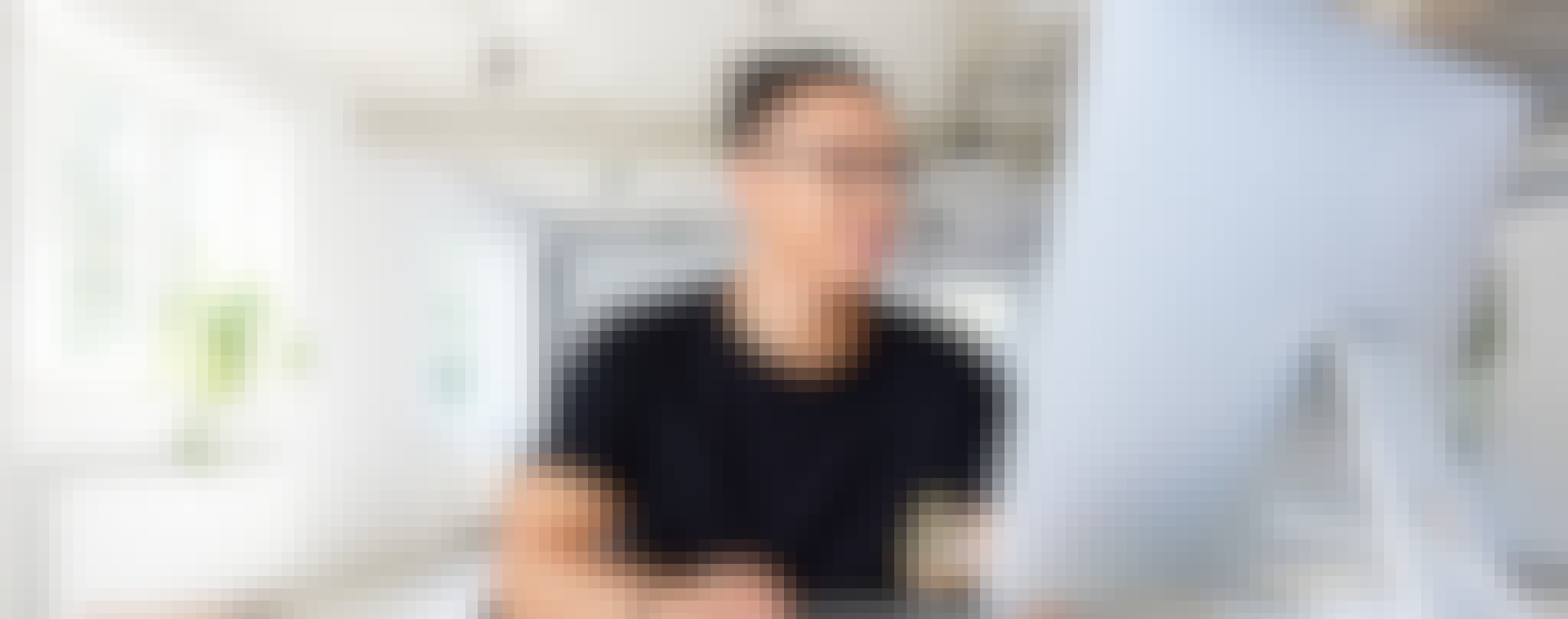
About this Guided Project
Learn step-by-step
In a video that plays in a split-screen with your work area, your instructor will walk you through these steps:
Import the data
Process the data
Regularization and Dropout
Creating the Experiment
Assess the final results
Recommended experience
Python programming experience and a basic understanding of neural networks is recommended.
6 project images
Instructor

Offered by
How you'll learn
Skill-based, hands-on learning
Practice new skills by completing job-related tasks.
Expert guidance
Follow along with pre-recorded videos from experts using a unique side-by-side interface.
No downloads or installation required
Access the tools and resources you need in a pre-configured cloud workspace.
Available only on desktop
This Guided Project is designed for laptops or desktop computers with a reliable Internet connection, not mobile devices.
Why people choose Coursera for their career




Learner reviews
Showing 3 of 76
76 reviews
- 5 stars
83.11%
- 4 stars
14.28%
- 3 stars
1.29%
- 2 stars
0%
- 1 star
1.29%
New to Machine Learning? Start here.

Open new doors with Coursera Plus
Unlimited access to 7,000+ world-class courses, hands-on projects, and job-ready certificate programs - all included in your subscription
Advance your career with an online degree
Earn a degree from world-class universities - 100% online
Join over 3,400 global companies that choose Coursera for Business
Upskill your employees to excel in the digital economy
Frequently asked questions
By purchasing a Guided Project, you'll get everything you need to complete the Guided Project including access to a cloud desktop workspace through your web browser that contains the files and software you need to get started, plus step-by-step video instruction from a subject matter expert.
Because your workspace contains a cloud desktop that is sized for a laptop or desktop computer, Guided Projects are not available on your mobile device.
Guided Project instructors are subject matter experts who have experience in the skill, tool or domain of their project and are passionate about sharing their knowledge to impact millions of learners around the world.