This IBM short course, a part of Generative AI Engineering Essentials with LLMs Professional Certificate, will teach you the basics of using generative AI and Large Language Models (LLMs). This course is suitable for existing and aspiring data scientists, machine learning engineers, deep-learning engineers, and AI engineers.

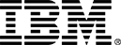
Generative AI and LLMs: Architecture and Data Preparation
Taught in English
Some content may not be translated
Recommended experience
What you'll learn
Differentiate between generative AI architectures and models, such as RNNs, Transformers, VAEs, GANs, and Diffusion Models.
Describe how LLMs, such as GPT, BERT, BART, and T5, are used in language processing.
Implement tokenization to preprocess raw textual data using NLP libraries such as NLTK, spaCy, BertTokenizer, and XLNetTokenizer.
Create an NLP data loader using PyTorch to perform tokenization, numericalization, and padding of text data.
Details to know

Add to your LinkedIn profile
May 2024
4 assignments
See how employees at top companies are mastering in-demand skills
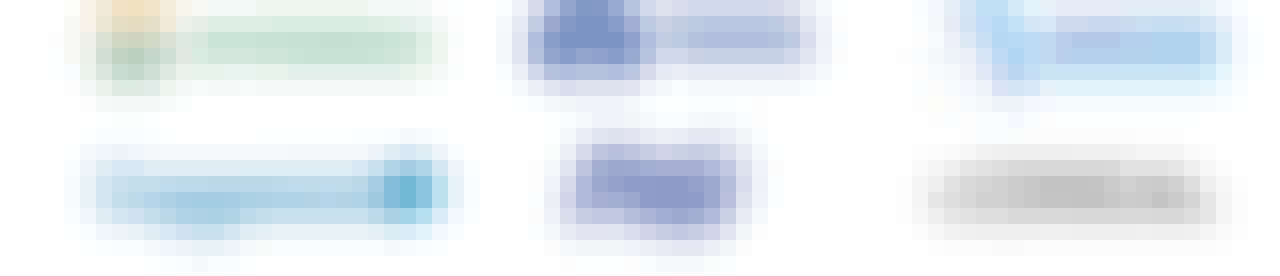
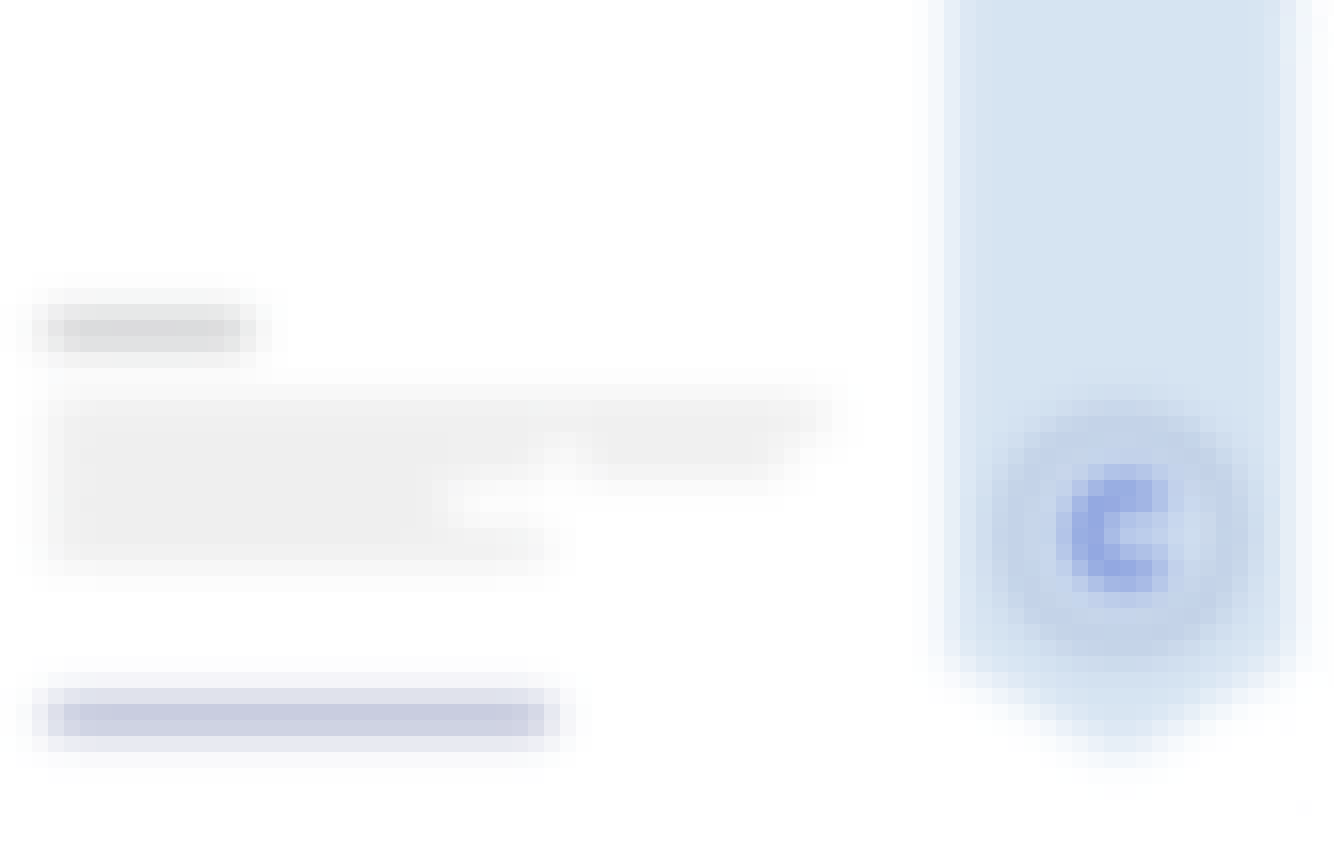
Earn a career certificate
Add this credential to your LinkedIn profile, resume, or CV
Share it on social media and in your performance review
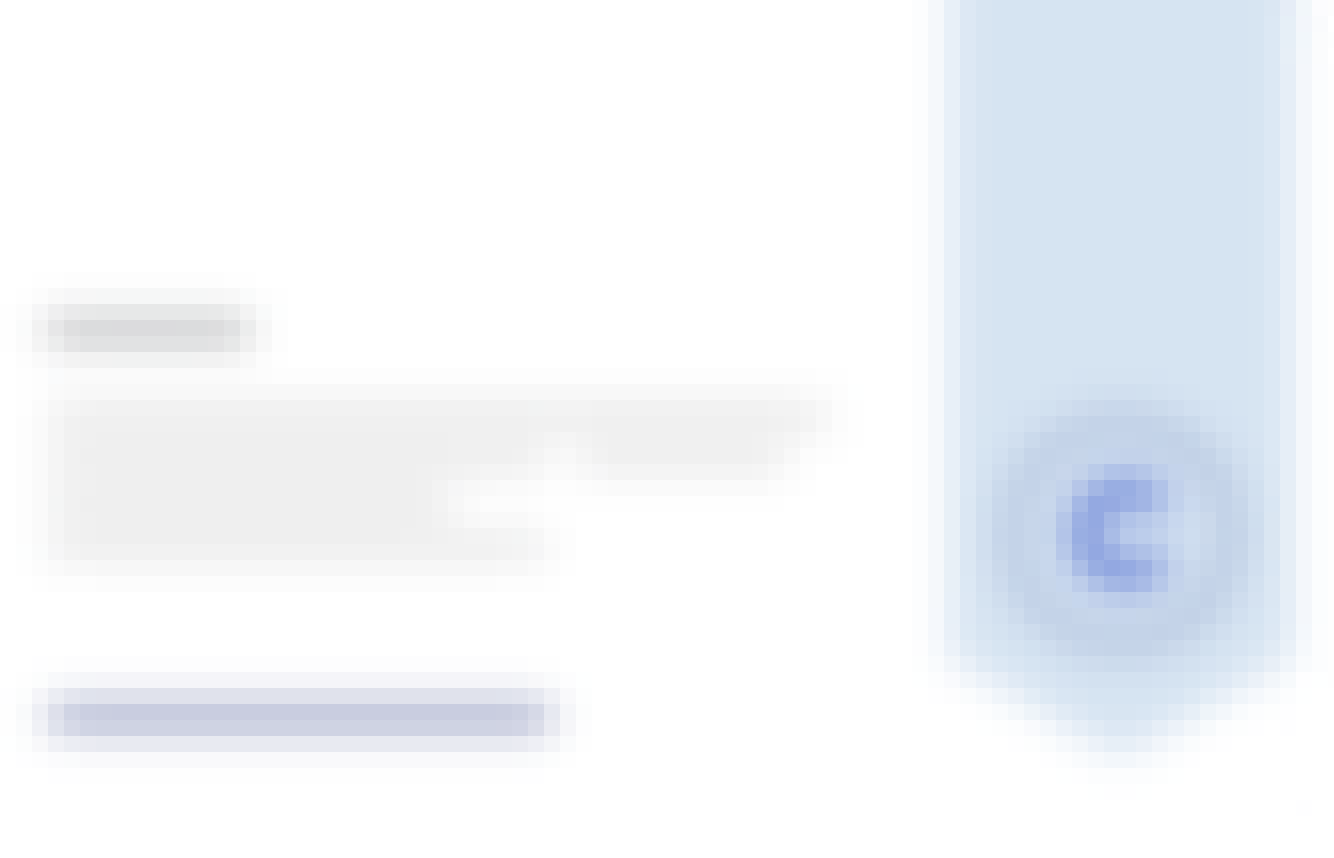
There are 2 modules in this course
In this module, you will learn about the significance of generative AI models and how they are used across a wide range of fields for generating various types of content. You will learn about the architectures and models commonly used in generative AI and the differences in the training approaches of these models. You will learn how large language models (LLMs) are used to build NLP-based applications. You will build a simple chatbot using the transformers library from Hugging Face.
What's included
4 videos2 readings2 assignments1 app item3 plugins
In this module, you will learn to prepare data for training large language models (LLMs) by implementing tokenization. You will learn about the tokenization methods and the use of tokenizers. You will also learn about the purpose of data loaders and how you can use the DataLoader class in PyTorch. You will implement tokenization using various libraries such as nltk, spaCy, BertTokenizer, and XLNetTokenizer. You will also create a data loader with a collate function that processes batches of text.
What's included
2 videos4 readings2 assignments2 app items2 plugins
Offered by
Recommended if you're interested in Machine Learning
Why people choose Coursera for their career




New to Machine Learning? Start here.

Open new doors with Coursera Plus
Unlimited access to 7,000+ world-class courses, hands-on projects, and job-ready certificate programs - all included in your subscription
Advance your career with an online degree
Earn a degree from world-class universities - 100% online
Join over 3,400 global companies that choose Coursera for Business
Upskill your employees to excel in the digital economy
Frequently asked questions
It will take only two weeks to complete this course if you spend two hours of study time per week.
It will be good if you have a basic knowledge of Python and PyTorch and a familiarity with machine learning and neural network concepts.
This course is part of a specialization. When you complete the specialization, you will prepare yourself with the skills and confidence to take on jobs such as AI Engineer, NLP Engineer, Machine Learning Engineer, Deep Learning Engineer, and Data Scientist.