In this project, learners will gain the skill of building and evaluating machine learning models using TensorFlow Decision Forests to accurately classify penguin species based on physical measurements. They will construct a comprehensive machine learning model under the guidance of the instructor. Learners will master specific skills including data preprocessing and cleaning, feature selection and importance analysis, and model evaluation using performance metrics. These skills will enable learners to handle real-world data challenges effectively. The benefit of taking this project is that it provides practical, hands-on experience in applying machine learning techniques to a real-world dataset, enhancing learners' ability to develop accurate and reliable models for ecological and conservation purposes. This project is suitable for TensorFlow beginners with a decent Python background, including knowledge of classes, functions, and some experience with pandas or numpy. While conceptual knowledge related to decision trees and random forests would be helpful, it is not required.

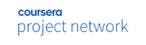
TensorFlow Prediction: Identify Penguin Species
Taught in English

Instructor: Christopher Smyth
Included with
Guided Project
Recommended experience
What you'll learn
Transform a dataset into TensorFlow-compatible format for optimized training and testing within TensorFlow environments.
Train a TensorFlow Decision Forest Model.
Evaluate a TensorFlow Decision Forest Model using standard metrics and data splits.
Skills you'll practice
Details to know

Add to your LinkedIn profile
May 2024
Guided Project
Recommended experience
See how employees at top companies are mastering in-demand skills
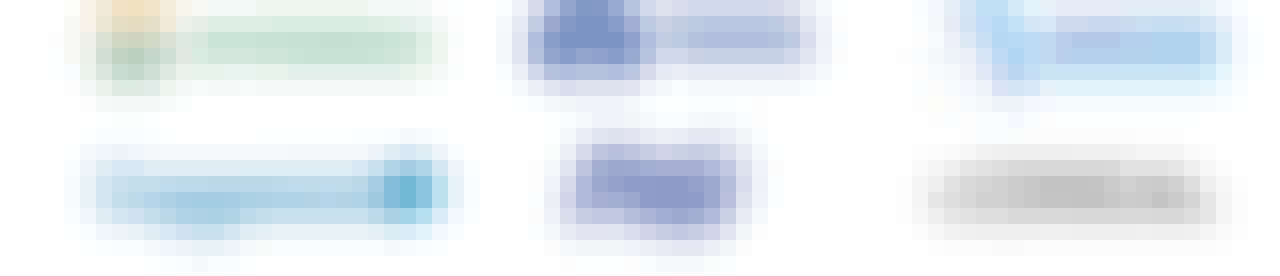
Learn, practice, and apply job-ready skills in less than 2 hours
- Receive training from industry experts
- Gain hands-on experience solving real-world job tasks
- Build confidence using the latest tools and technologies
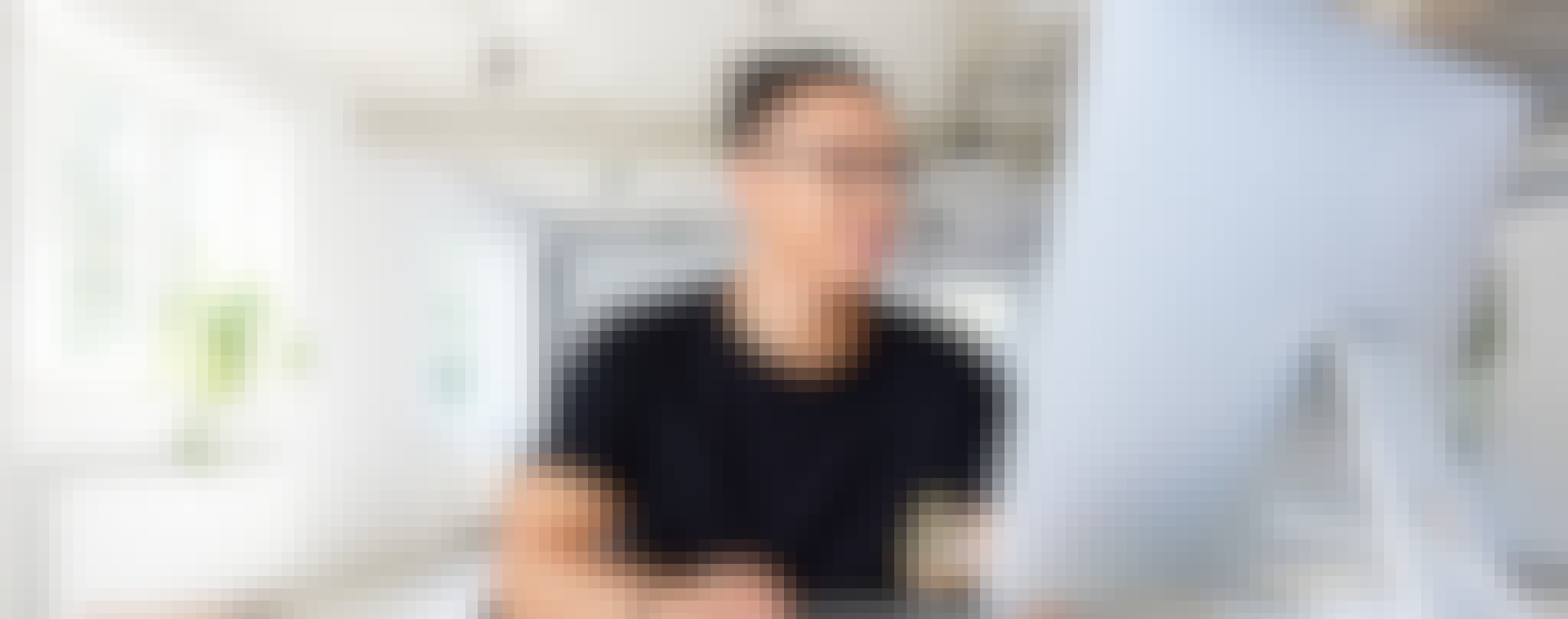
About this Guided Project
Learn step-by-step
In a video that plays in a split-screen with your work area, your instructor will walk you through these steps:
Install TensorFlow Decision Forests
Load and Transform the Penguins Dataset
Split Data into Test and Training Datasets and Prepare Data for TensorFlow
Train and Evaluate Random Forest Model
Evaluate TensorFlow Random Forest Model Against Test Data
Recommended experience
Need Python programming (functions, loops), Pandas dataframes. Machine learning background (training procedure, random forests) would be nice to have
1 project image
Instructor

Offered by
How you'll learn
Skill-based, hands-on learning
Practice new skills by completing job-related tasks.
Expert guidance
Follow along with pre-recorded videos from experts using a unique side-by-side interface.
No downloads or installation required
Access the tools and resources you need in a pre-configured cloud workspace.
Available only on desktop
This Guided Project is designed for laptops or desktop computers with a reliable Internet connection, not mobile devices.
Why people choose Coursera for their career




New to Data Analysis? Start here.

Open new doors with Coursera Plus
Unlimited access to 7,000+ world-class courses, hands-on projects, and job-ready certificate programs - all included in your subscription
Advance your career with an online degree
Earn a degree from world-class universities - 100% online
Join over 3,400 global companies that choose Coursera for Business
Upskill your employees to excel in the digital economy
Frequently asked questions
By purchasing a Guided Project, you'll get everything you need to complete the Guided Project including access to a cloud desktop workspace through your web browser that contains the files and software you need to get started, plus step-by-step video instruction from a subject matter expert.
Because your workspace contains a cloud desktop that is sized for a laptop or desktop computer, Guided Projects are not available on your mobile device.
Guided Project instructors are subject matter experts who have experience in the skill, tool or domain of their project and are passionate about sharing their knowledge to impact millions of learners around the world.