Probabilistic graphical models (PGMs) are a rich framework for encoding probability distributions over complex domains: joint (multivariate) distributions over large numbers of random variables that interact with each other. These representations sit at the intersection of statistics and computer science, relying on concepts from probability theory, graph algorithms, machine learning, and more. They are the basis for the state-of-the-art methods in a wide variety of applications, such as medical diagnosis, image understanding, speech recognition, natural language processing, and many, many more. They are also a foundational tool in formulating many machine learning problems.

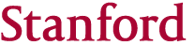
Probabilistic Graphical Models 3: Learning
This course is part of Probabilistic Graphical Models Specialization
Taught in English
Some content may not be translated

Instructor: Daphne Koller
21,484 already enrolled
Course
(298 reviews)
Skills you'll gain
Details to know

Add to your LinkedIn profile
8 quizzes
Course
(298 reviews)
See how employees at top companies are mastering in-demand skills
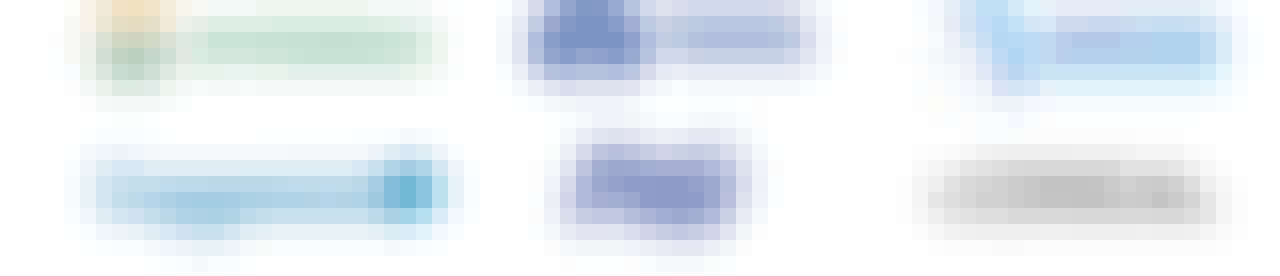
Build your subject-matter expertise
- Learn new concepts from industry experts
- Gain a foundational understanding of a subject or tool
- Develop job-relevant skills with hands-on projects
- Earn a shareable career certificate
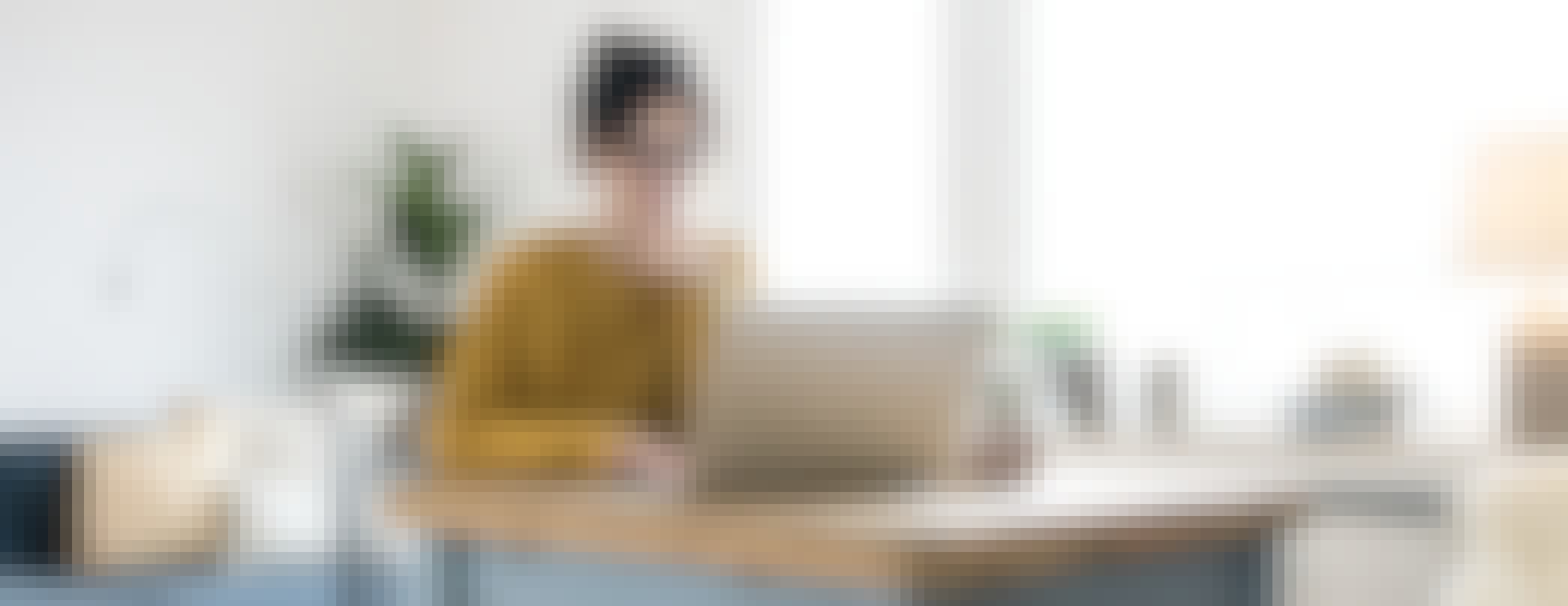
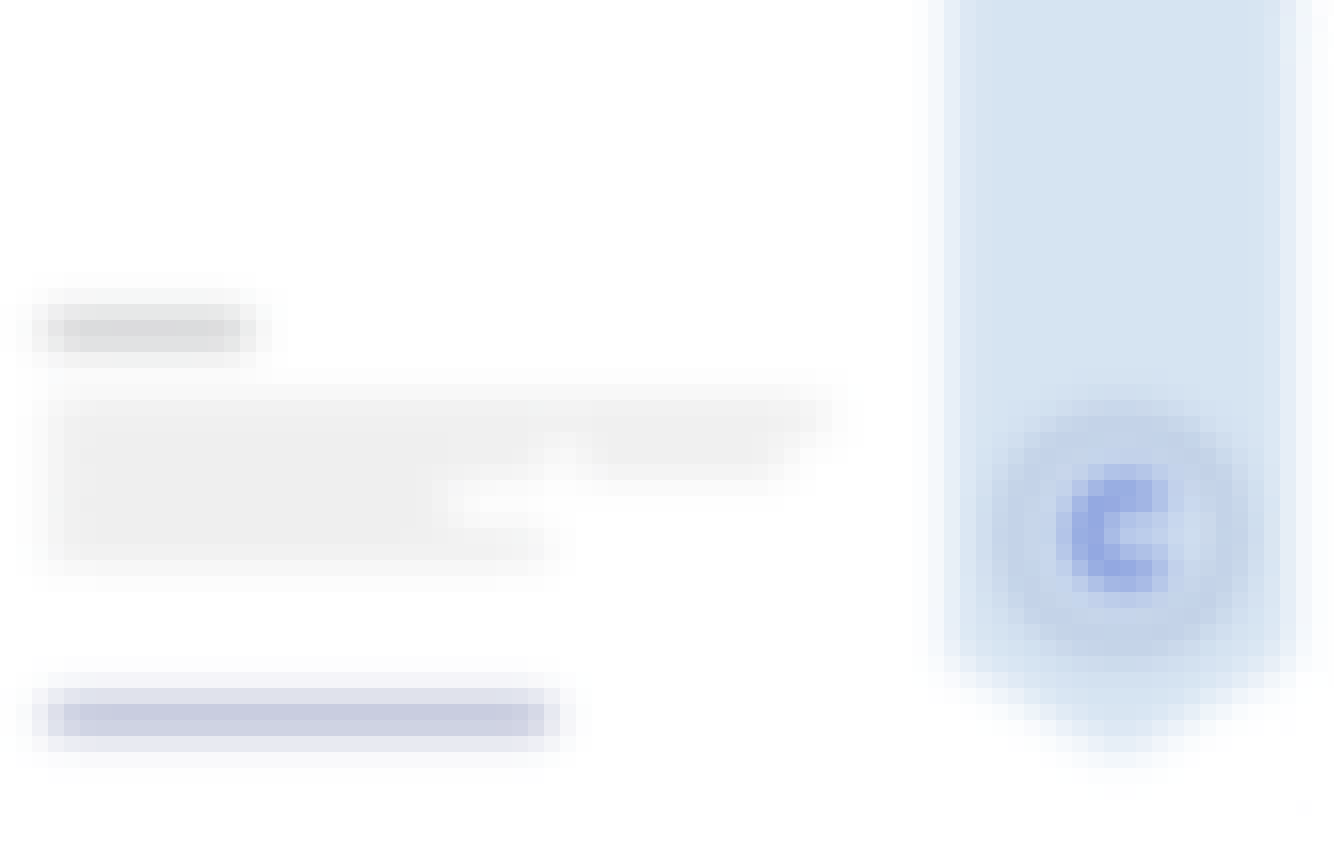
Earn a career certificate
Add this credential to your LinkedIn profile, resume, or CV
Share it on social media and in your performance review
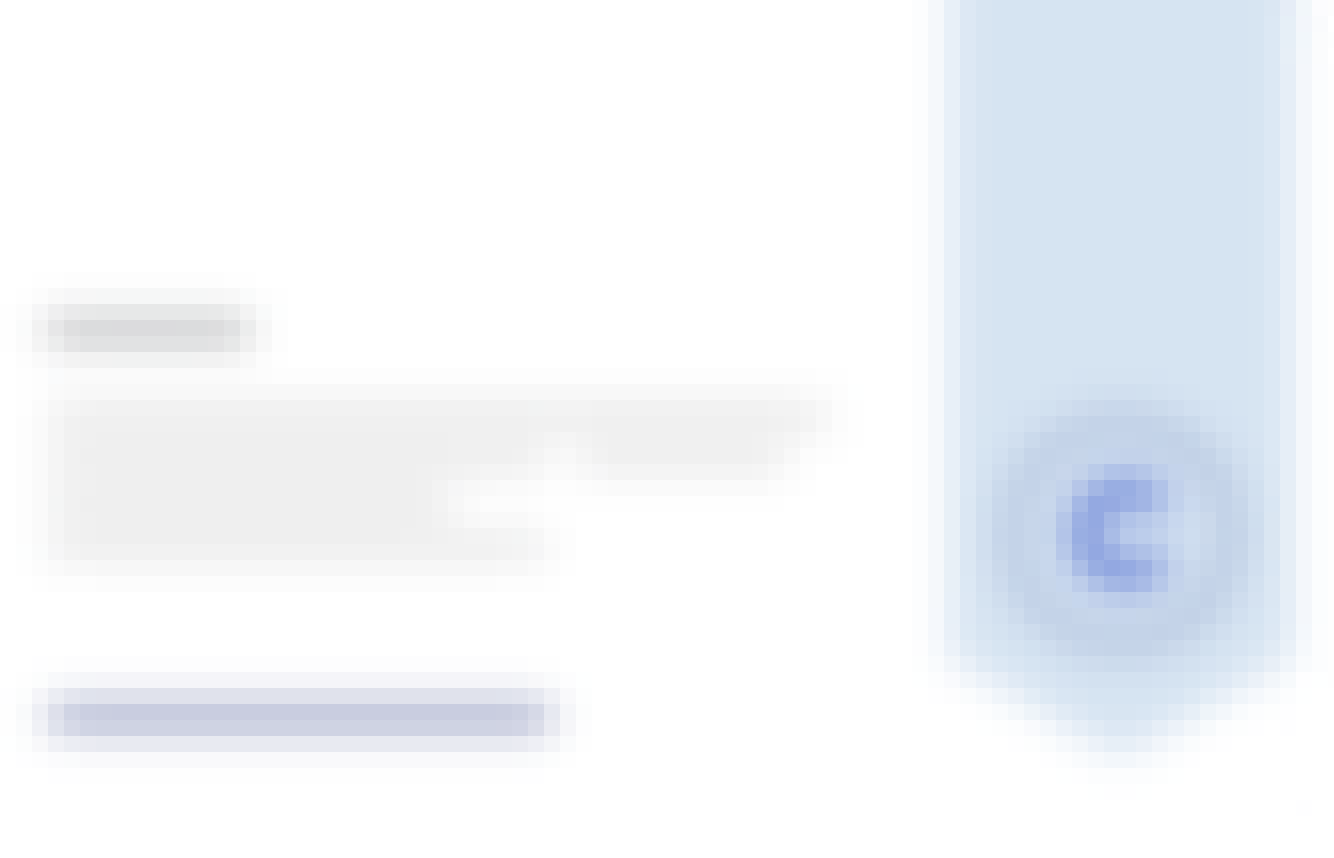
There are 8 modules in this course
This module presents some of the learning tasks for probabilistic graphical models that we will tackle in this course.
What's included
1 video
This module contains some basic concepts from the general framework of machine learning, taken from Professor Andrew Ng's Stanford class offered on Coursera. Many of these concepts are highly relevant to the problems we'll tackle in this course.
What's included
6 videos
This module discusses the simples and most basic of the learning problems in probabilistic graphical models: that of parameter estimation in a Bayesian network. We discuss maximum likelihood estimation, and the issues with it. We then discuss Bayesian estimation and how it can ameliorate these problems.
What's included
5 videos2 quizzes
In this module, we discuss the parameter estimation problem for Markov networks - undirected graphical models. This task is considerably more complex, both conceptually and computationally, than parameter estimation for Bayesian networks, due to the issues presented by the global partition function.
What's included
3 videos1 quiz1 programming assignment
This module discusses the problem of learning the structure of Bayesian networks. We first discuss how this problem can be formulated as an optimization problem over a space of graph structures, and what are good ways to score different structures so as to trade off fit to data and model complexity. We then talk about how the optimization problem can be solved: exactly in a few cases, approximately in most others.
What's included
7 videos2 quizzes1 programming assignment
In this module, we discuss the problem of learning models in cases where some of the variables in some of the data cases are not fully observed. We discuss why this situation is considerably more complex than the fully observable case. We then present the Expectation Maximization (EM) algorithm, which is used in a wide variety of problems.
What's included
5 videos2 quizzes1 programming assignment
This module summarizes some of the issues that arise when learning probabilistic graphical models from data. It also contains the course final.
What's included
1 video1 quiz
This module contains an overview of PGM methods as a whole, discussing some of the real-world tradeoffs when using this framework in practice. It refers to topics from all three of the PGM courses.
What's included
1 video
Instructor

Offered by
Recommended if you're interested in Machine Learning
Stanford University
Stanford University
Databricks
Duke University
Why people choose Coursera for their career




Learner reviews
Showing 3 of 298
298 reviews
- 5 stars
71.14%
- 4 stars
19.46%
- 3 stars
5.36%
- 2 stars
3.02%
- 1 star
1%
New to Machine Learning? Start here.

Open new doors with Coursera Plus
Unlimited access to 7,000+ world-class courses, hands-on projects, and job-ready certificate programs - all included in your subscription
Advance your career with an online degree
Earn a degree from world-class universities - 100% online
Join over 3,400 global companies that choose Coursera for Business
Upskill your employees to excel in the digital economy
Frequently asked questions
Compute the sufficient statistics of a data set that are necessary for learning a PGM from data
Implement both maximum likelihood and Bayesian parameter estimation for Bayesian networks
Implement maximum likelihood and MAP parameter estimation for Markov networks
Formulate a structure learning problem as a combinatorial optimization task over a space of network structure, and evaluate which scoring function is appropriate for a given situation
Utilize PGM inference algorithms in ways that support more effective parameter estimation for PGMs
Implement the Expectation Maximization (EM) algorithm for Bayesian networks
Honors track learners will get hands-on experience in implementing both EM and structure learning for tree-structured networks, and apply them to real-world tasks
Access to lectures and assignments depends on your type of enrollment. If you take a course in audit mode, you will be able to see most course materials for free. To access graded assignments and to earn a Certificate, you will need to purchase the Certificate experience, during or after your audit. If you don't see the audit option:
The course may not offer an audit option. You can try a Free Trial instead, or apply for Financial Aid.
The course may offer 'Full Course, No Certificate' instead. This option lets you see all course materials, submit required assessments, and get a final grade. This also means that you will not be able to purchase a Certificate experience.
When you enroll in the course, you get access to all of the courses in the Specialization, and you earn a certificate when you complete the work. Your electronic Certificate will be added to your Accomplishments page - from there, you can print your Certificate or add it to your LinkedIn profile. If you only want to read and view the course content, you can audit the course for free.