In this course, you will learn how to solve problems with large, high-dimensional, and potentially infinite state spaces. You will see that estimating value functions can be cast as a supervised learning problem---function approximation---allowing you to build agents that carefully balance generalization and discrimination in order to maximize reward. We will begin this journey by investigating how our policy evaluation or prediction methods like Monte Carlo and TD can be extended to the function approximation setting. You will learn about feature construction techniques for RL, and representation learning via neural networks and backprop. We conclude this course with a deep-dive into policy gradient methods; a way to learn policies directly without learning a value function. In this course you will solve two continuous-state control tasks and investigate the benefits of policy gradient methods in a continuous-action environment.

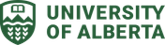
Prediction and Control with Function Approximation
This course is part of Reinforcement Learning Specialization
Taught in English
Some content may not be translated


Instructors: Martha White
24,982 already enrolled
Included with
Course
(816 reviews)
90%
Recommended experience
Skills you'll gain
Details to know

Add to your LinkedIn profile
4 quizzes
Course
(816 reviews)
90%
Recommended experience
See how employees at top companies are mastering in-demand skills
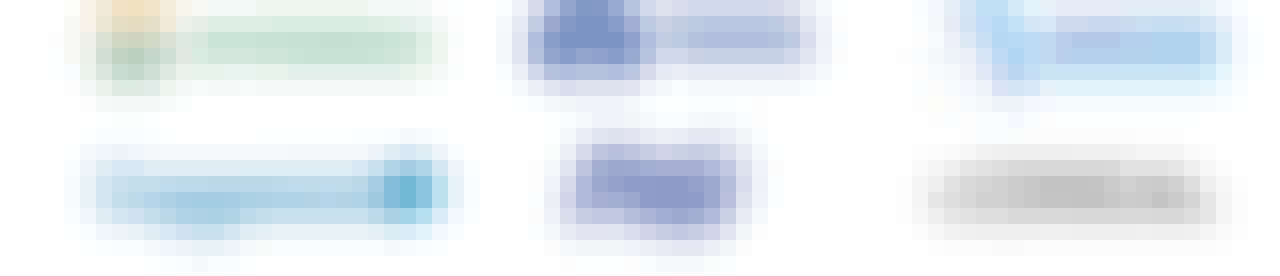
Build your subject-matter expertise
- Learn new concepts from industry experts
- Gain a foundational understanding of a subject or tool
- Develop job-relevant skills with hands-on projects
- Earn a shareable career certificate
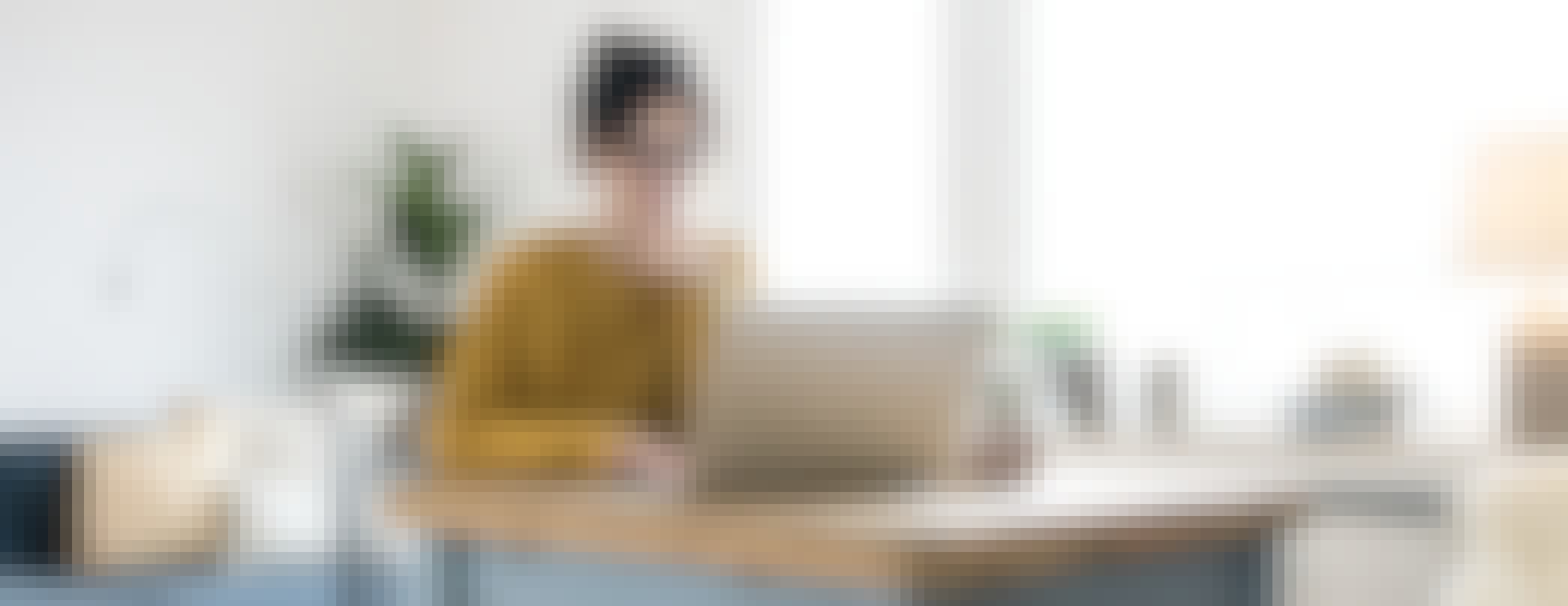
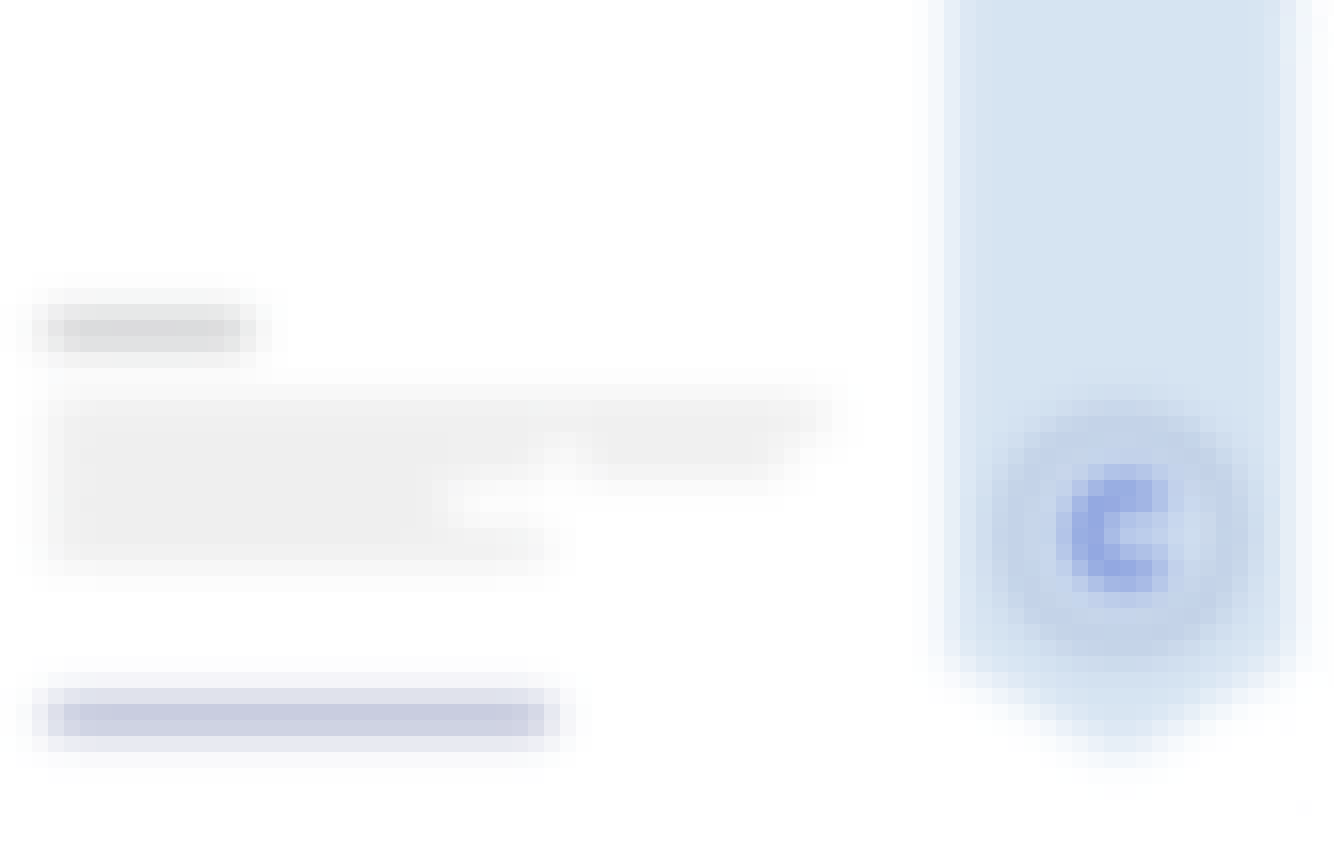
Earn a career certificate
Add this credential to your LinkedIn profile, resume, or CV
Share it on social media and in your performance review
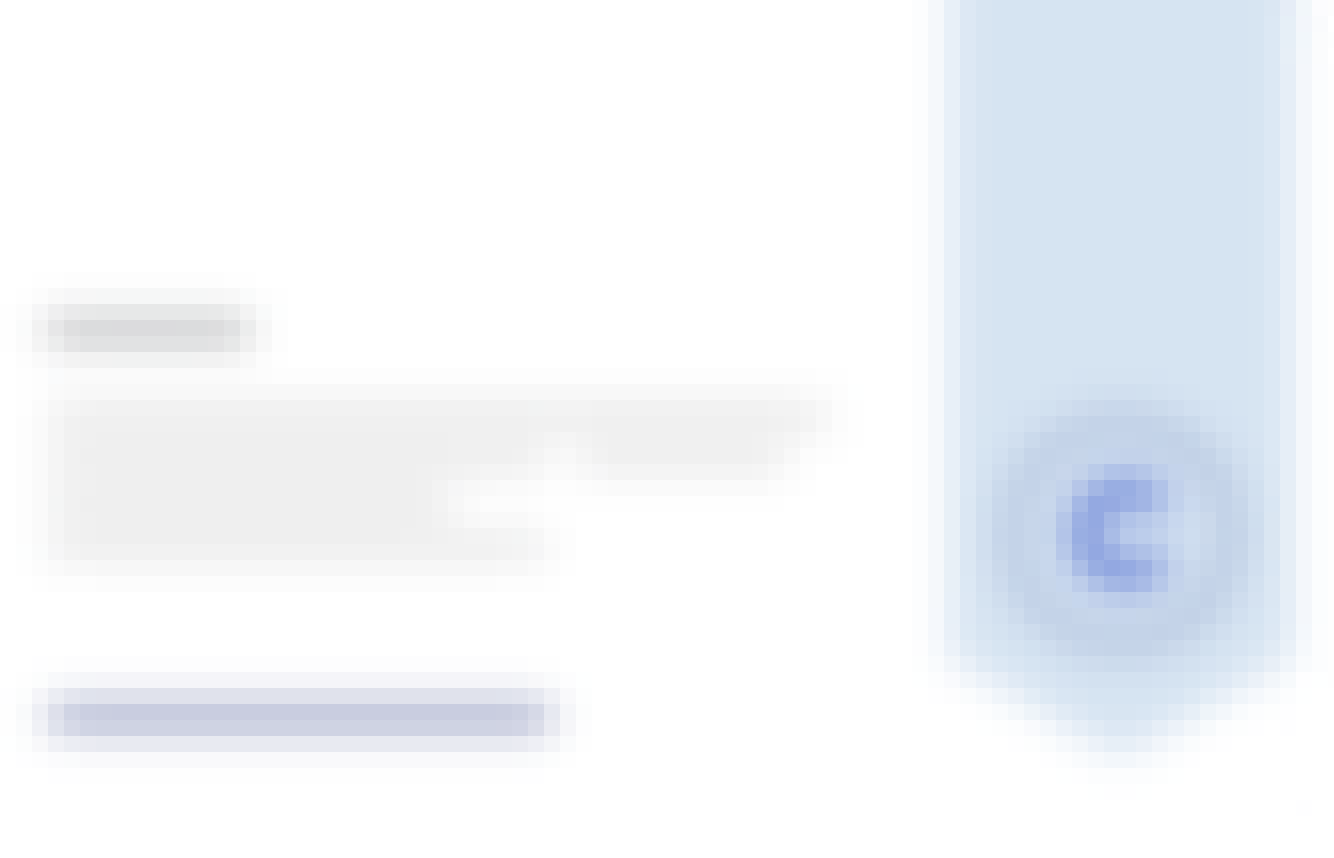
There are 5 modules in this course
Welcome to the third course in the Reinforcement Learning Specialization: Prediction and Control with Function Approximation, brought to you by the University of Alberta, Onlea, and Coursera. In this pre-course module, you'll be introduced to your instructors, and get a flavour of what the course has in store for you. Make sure to introduce yourself to your classmates in the "Meet and Greet" section!
What's included
2 videos2 readings1 discussion prompt
This week you will learn how to estimate a value function for a given policy, when the number of states is much larger than the memory available to the agent. You will learn how to specify a parametric form of the value function, how to specify an objective function, and how estimating gradient descent can be used to estimate values from interaction with the world.
What's included
13 videos2 readings1 quiz1 programming assignment1 discussion prompt
The features used to construct the agent’s value estimates are perhaps the most crucial part of a successful learning system. In this module we discuss two basic strategies for constructing features: (1) fixed basis that form an exhaustive partition of the input, and (2) adapting the features while the agent interacts with the world via Neural Networks and Backpropagation. In this week’s graded assessment you will solve a simple but infinite state prediction task with a Neural Network and TD learning.
What's included
11 videos2 readings1 quiz1 programming assignment1 discussion prompt
This week, you will see that the concepts and tools introduced in modules two and three allow straightforward extension of classic TD control methods to the function approximation setting. In particular, you will learn how to find the optimal policy in infinite-state MDPs by simply combining semi-gradient TD methods with generalized policy iteration, yielding classic control methods like Q-learning, and Sarsa. We conclude with a discussion of a new problem formulation for RL---average reward---which will undoubtedly be used in many applications of RL in the future.
What's included
7 videos2 readings1 quiz1 programming assignment2 discussion prompts
Every algorithm you have learned about so far estimates a value function as an intermediate step towards the goal of finding an optimal policy. An alternative strategy is to directly learn the parameters of the policy. This week you will learn about these policy gradient methods, and their advantages over value-function based methods. You will also learn how policy gradient methods can be used to find the optimal policy in tasks with both continuous state and action spaces.
What's included
11 videos2 readings1 quiz1 programming assignment1 discussion prompt
Instructors


Recommended if you're interested in Machine Learning
University of Alberta
University of Alberta
University of Alberta
Coursera Project Network
Why people choose Coursera for their career




Learner reviews
Showing 3 of 816
816 reviews
- 5 stars
84.59%
- 4 stars
12.34%
- 3 stars
1.95%
- 2 stars
0.73%
- 1 star
0.36%
New to Machine Learning? Start here.

Open new doors with Coursera Plus
Unlimited access to 7,000+ world-class courses, hands-on projects, and job-ready certificate programs - all included in your subscription
Advance your career with an online degree
Earn a degree from world-class universities - 100% online
Join over 3,400 global companies that choose Coursera for Business
Upskill your employees to excel in the digital economy
Frequently asked questions
Access to lectures and assignments depends on your type of enrollment. If you take a course in audit mode, you will be able to see most course materials for free. To access graded assignments and to earn a Certificate, you will need to purchase the Certificate experience, during or after your audit. If you don't see the audit option:
The course may not offer an audit option. You can try a Free Trial instead, or apply for Financial Aid.
The course may offer 'Full Course, No Certificate' instead. This option lets you see all course materials, submit required assessments, and get a final grade. This also means that you will not be able to purchase a Certificate experience.
When you enroll in the course, you get access to all of the courses in the Specialization, and you earn a certificate when you complete the work. Your electronic Certificate will be added to your Accomplishments page - from there, you can print your Certificate or add it to your LinkedIn profile. If you only want to read and view the course content, you can audit the course for free.
If you subscribed, you get a 7-day free trial during which you can cancel at no penalty. After that, we don’t give refunds, but you can cancel your subscription at any time. See our full refund policy.