This course, which is designed to serve as the first course in the Recommender Systems specialization, introduces the concept of recommender systems, reviews several examples in detail, and leads you through non-personalized recommendation using summary statistics and product associations, basic stereotype-based or demographic recommendations, and content-based filtering recommendations.

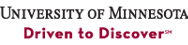
Introduction to Recommender Systems: Non-Personalized and Content-Based
This course is part of Recommender Systems Specialization
Taught in English
Some content may not be translated


Instructors: Joseph A Konstan
38,284 already enrolled
Included with
Course
(641 reviews)
89%
Skills you'll gain
Details to know

Add to your LinkedIn profile
12 quizzes
Course
(641 reviews)
89%
See how employees at top companies are mastering in-demand skills
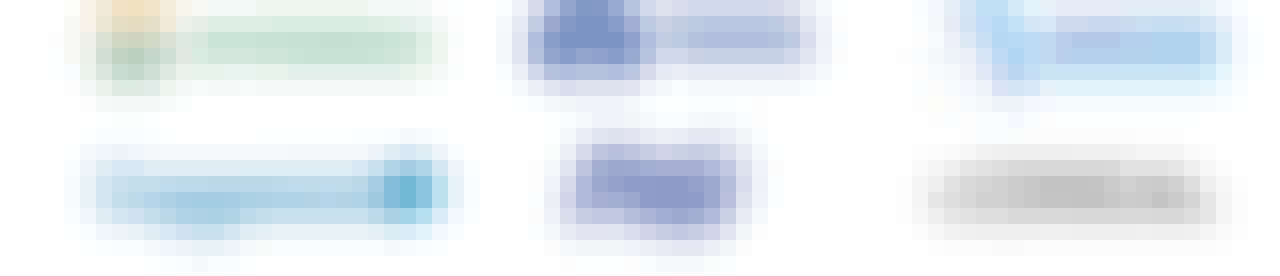
Build your subject-matter expertise
- Learn new concepts from industry experts
- Gain a foundational understanding of a subject or tool
- Develop job-relevant skills with hands-on projects
- Earn a shareable career certificate
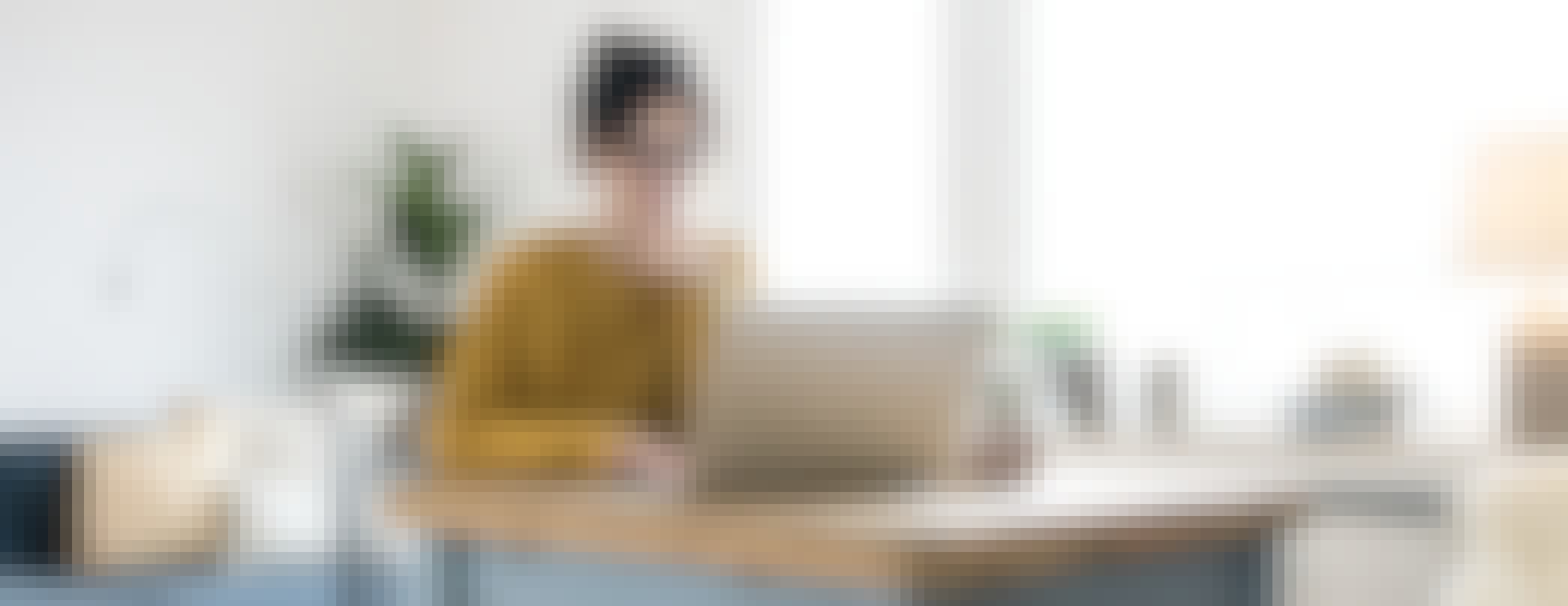
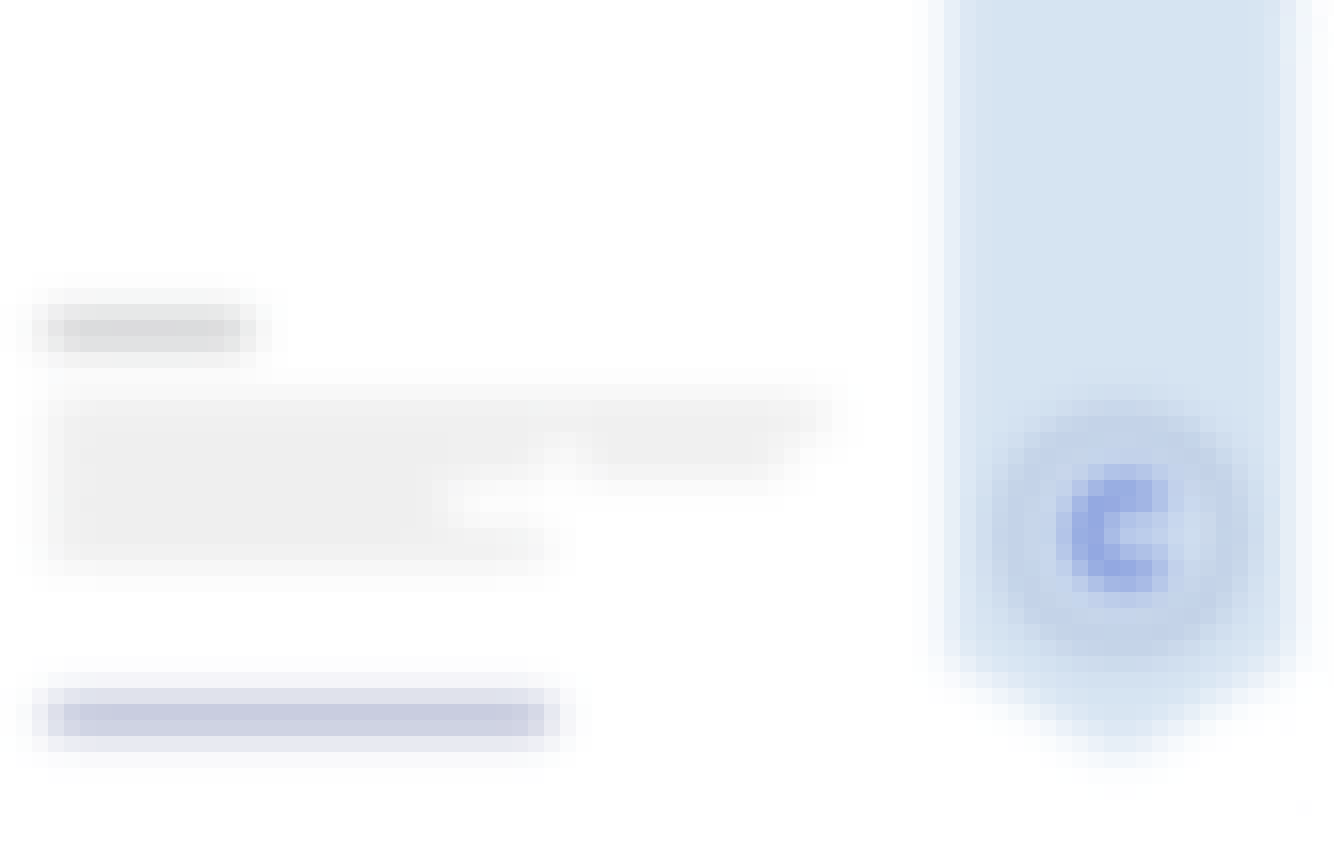
Earn a career certificate
Add this credential to your LinkedIn profile, resume, or CV
Share it on social media and in your performance review
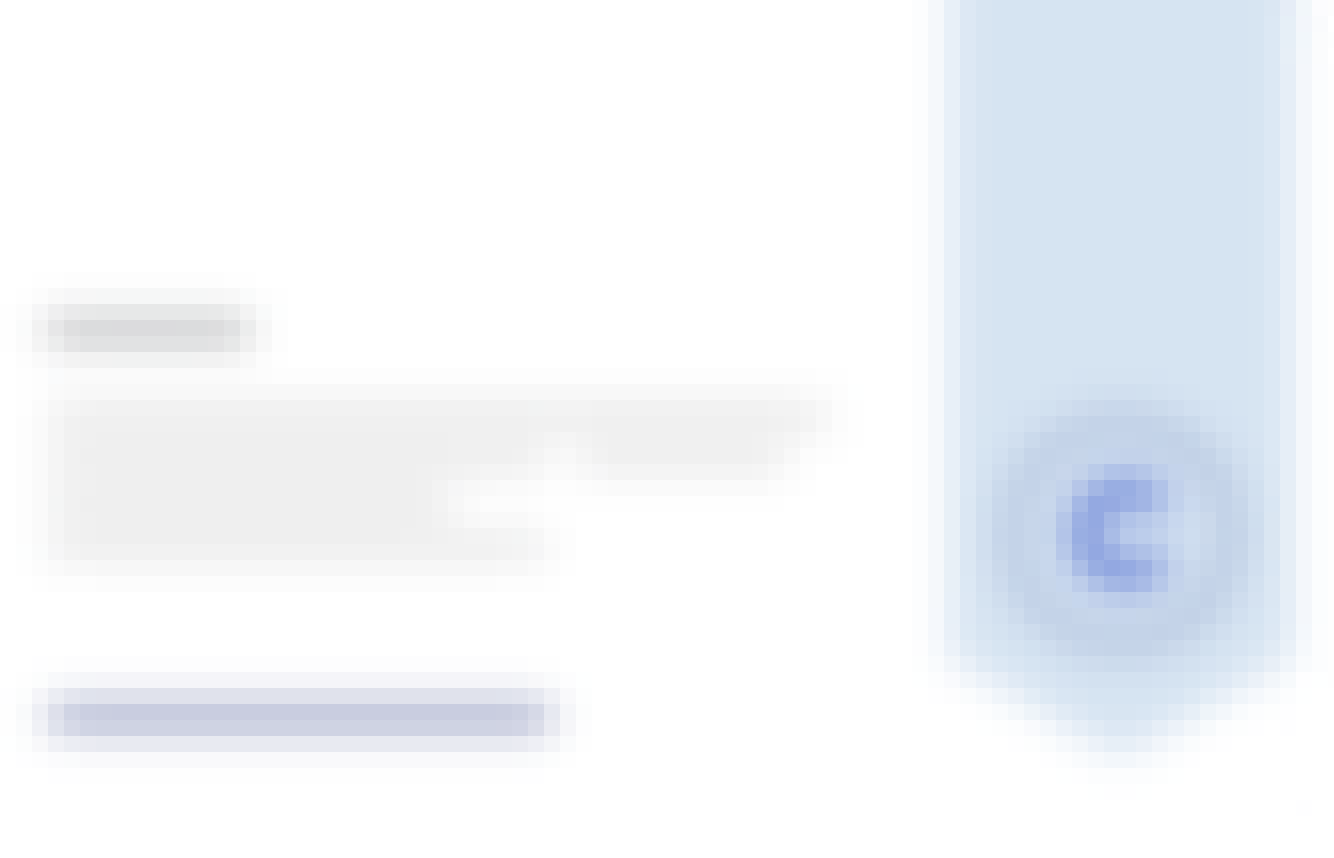
There are 6 modules in this course
This brief module introduces the topic of recommender systems (including placing the technology in historical context) and provides an overview of the structure and coverage of the course and specialization.
What's included
2 videos1 reading
This module introduces recommender systems in more depth. It includes a detailed taxonomy of the types of recommender systems, and also includes tours of two systems heavily dependent on recommender technology: MovieLens and Amazon.com. There is an introductory assessment in the final lesson to ensure that you understand the core concepts behind recommendations before we start learning how to compute them.
What's included
9 videos2 readings2 quizzes
In this module, you will learn several techniques for non- and lightly-personalized recommendations, including how to use meaningful summary statistics, how to compute product association recommendations, and how to explore using demographics as a means for light personalization. There is both an assignment (trying out these techniques in a spreadsheet) and a quiz to test your comprehension.
What's included
7 videos5 readings8 quizzes1 programming assignment
The next topic in this course is content-based filtering, a technique for personalization based on building a profile of personal interests. Divided over two weeks, you will learn and practice the basic techniques for content-based filtering and then explore a variety of advanced interfaces and content-based computational techniques being used in recommender systems.
What's included
8 videos
The assessments for content-based filtering include an assignment where you compute three types of profile and prediction using a spreadsheet and a quiz on the topics covered. The assignment is in three parts -- a written assignment, a video intro, and a "quiz" where you provide answers from your work to be automatically graded.
What's included
2 videos3 readings2 quizzes1 programming assignment
We close this course with a set of mathematical notation that will be helpful as we move forward into a wider range of recommender systems (in later courses in this specialization).
What's included
2 videos1 reading
Instructors


Offered by
Recommended if you're interested in Machine Learning
University of Minnesota
University of Minnesota
EIT Digital
EIT Digital
Why people choose Coursera for their career




Learner reviews
Showing 3 of 641
641 reviews
- 5 stars
60.28%
- 4 stars
29.59%
- 3 stars
6.38%
- 2 stars
1.86%
- 1 star
1.86%
New to Machine Learning? Start here.

Open new doors with Coursera Plus
Unlimited access to 7,000+ world-class courses, hands-on projects, and job-ready certificate programs - all included in your subscription
Advance your career with an online degree
Earn a degree from world-class universities - 100% online
Join over 3,400 global companies that choose Coursera for Business
Upskill your employees to excel in the digital economy
Frequently asked questions
This specialization is a substantial extension and update of our original introductory course. It involves about 60% new and extended lectures and mostly new assignments and assessments. This course specifically has added material on stereotyped and demographic recommenders and on advanced techniques in content-based recommendation.
Access to lectures and assignments depends on your type of enrollment. If you take a course in audit mode, you will be able to see most course materials for free. To access graded assignments and to earn a Certificate, you will need to purchase the Certificate experience, during or after your audit. If you don't see the audit option:
The course may not offer an audit option. You can try a Free Trial instead, or apply for Financial Aid.
The course may offer 'Full Course, No Certificate' instead. This option lets you see all course materials, submit required assessments, and get a final grade. This also means that you will not be able to purchase a Certificate experience.
When you enroll in the course, you get access to all of the courses in the Specialization, and you earn a certificate when you complete the work. Your electronic Certificate will be added to your Accomplishments page - from there, you can print your Certificate or add it to your LinkedIn profile. If you only want to read and view the course content, you can audit the course for free.