This is the sixth of seven courses in the Google Advanced Data Analytics Certificate. In this course, you’ll learn about machine learning, which uses algorithms and statistics to teach computer systems to discover patterns in data. Data professionals use machine learning to help analyze large amounts of data, solve complex problems, and make accurate predictions. You’ll focus on the two main types of machine learning: supervised and unsupervised. You'll learn how to apply different machine learning models to business problems and become familiar with specific models such as Naive Bayes, decision tree, random forest, and more.

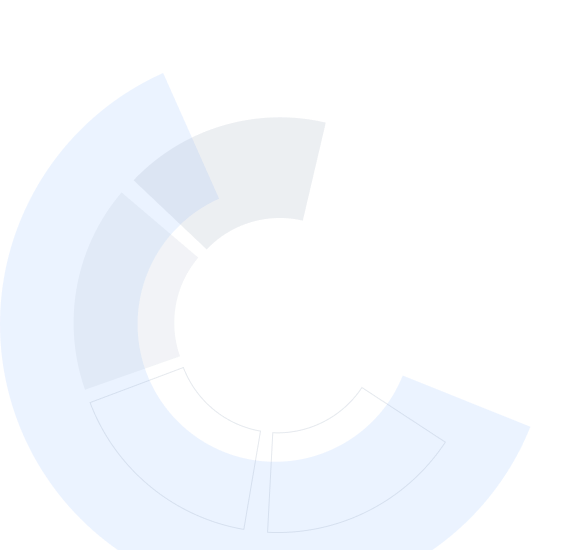
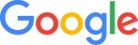
The Nuts and Bolts of Machine Learning
This course is part of Google Advanced Data Analytics Professional Certificate

Instructor: Google Career Certificates
Top Instructor
42,129 already enrolled
Included with
(402 reviews)
What you'll learn
Identify characteristics of the different types of machine learning
Prepare data for machine learning models
Build and evaluate supervised and unsupervised learning models using Python
Demonstrate proper model and metric selection for a machine learning algorithm
Skills you'll gain
Details to know

Add to your LinkedIn profile
22 quizzes
See how employees at top companies are mastering in-demand skills
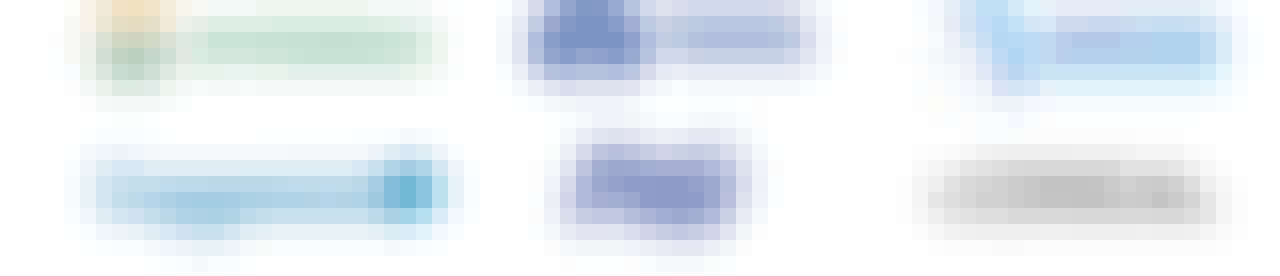
Build your Machine Learning expertise
- Learn new concepts from industry experts
- Gain a foundational understanding of a subject or tool
- Develop job-relevant skills with hands-on projects
- Earn a shareable career certificate from Google
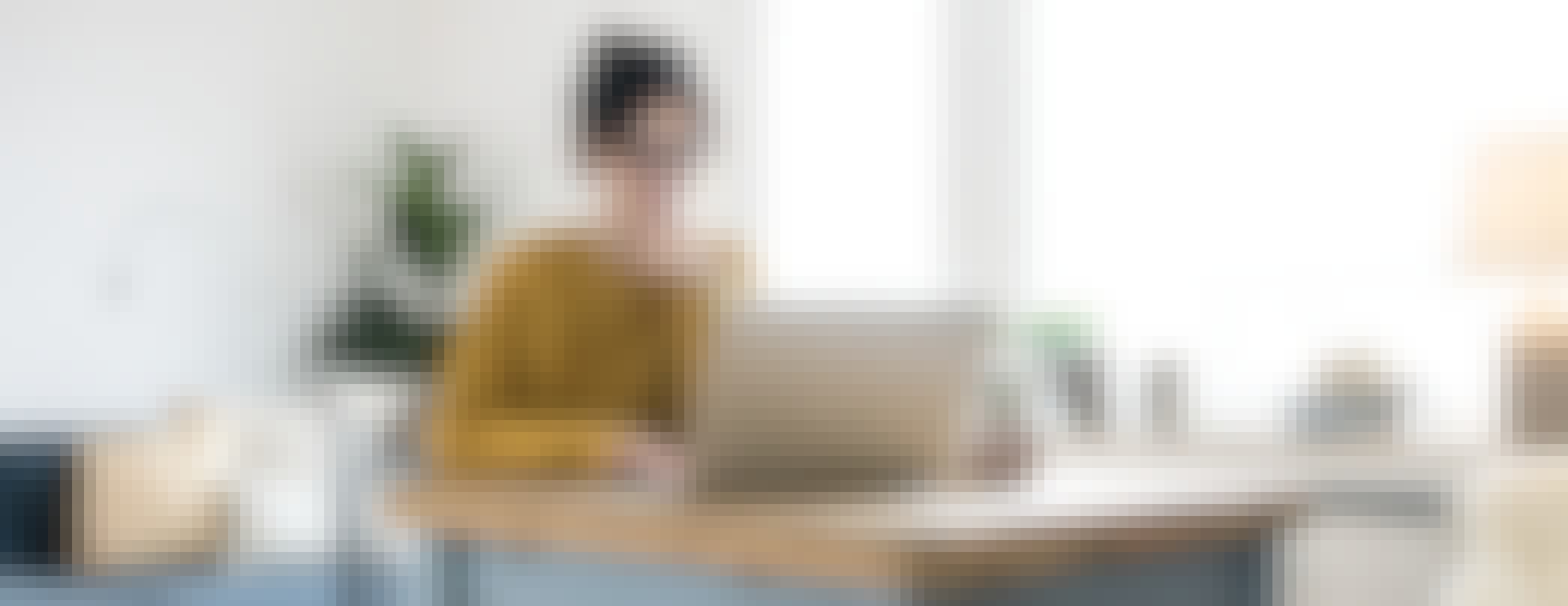
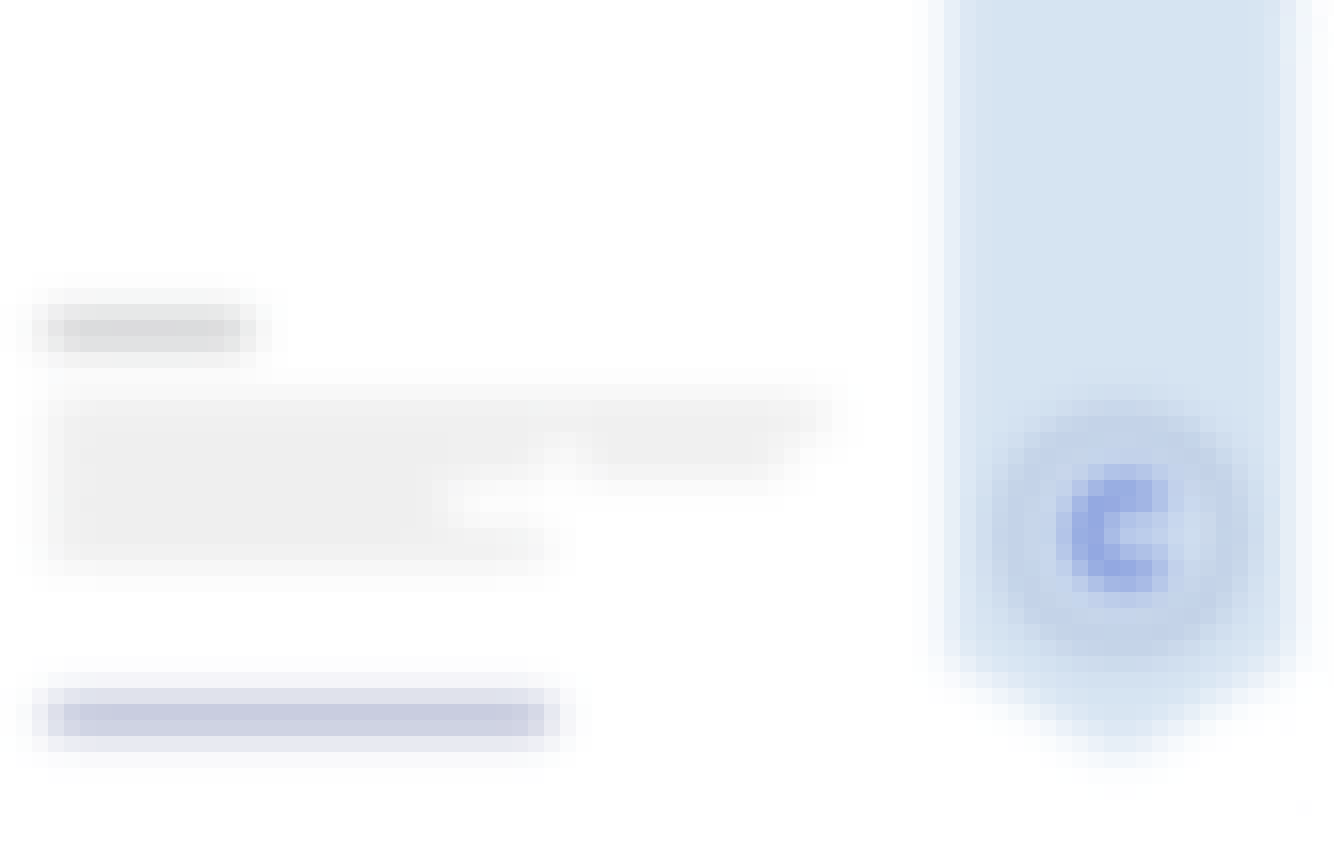
Earn a career certificate
Add this credential to your LinkedIn profile, resume, or CV
Share it on social media and in your performance review
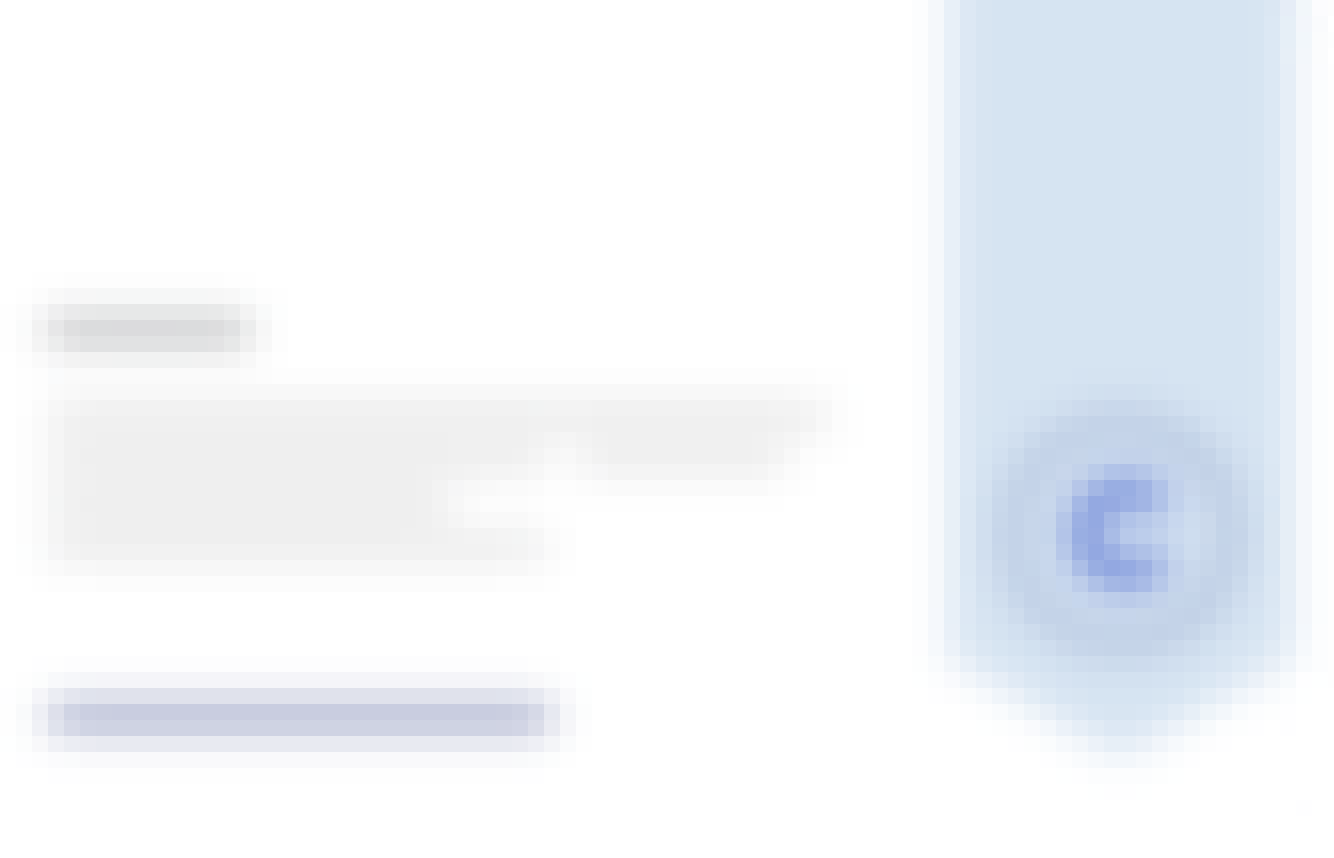
There are 5 modules in this course
You’ll start by exploring the basic concepts of machine learning and the role of machine learning in data science. Then, you’ll review the four main types of machine learning: supervised, unsupervised, reinforcement, and deep learning.
What's included
16 videos7 readings7 quizzes2 plugins
You’ll learn how data professionals use a structured workflow for machine learning. You'll identify the main steps of the workflow and the importance of each step in the overall process. Then, you'll learn how to apply specific machine learning models to business problems.
What's included
12 videos6 readings3 quizzes6 ungraded labs
You’ll learn more about one of the major types of machine learning: unsupervised learning. You'll begin by exploring the difference between supervised and unsupervised techniques and the benefits and uses of each approach. Then, you’ll learn how to apply two unsupervised machine learning models: clustering and K-means.
What's included
7 videos4 readings3 quizzes4 ungraded labs
Next, you’ll focus on supervised learning. You’ll learn how to test and validate the performance of supervised machine learning models such as decision tree, random forest, and gradient boosting.
What's included
16 videos11 readings5 quizzes10 ungraded labs1 plugin
You’ll complete the final end-of-course project by applying different machine learning models to a workplace scenario dataset.
What's included
5 videos10 readings4 quizzes6 ungraded labs
Instructor

Offered by
Recommended if you're interested in Machine Learning
Why people choose Coursera for their career




Learner reviews
Showing 3 of 402
402 reviews
- 5 stars
87.59%
- 4 stars
8.68%
- 3 stars
2.48%
- 2 stars
0.49%
- 1 star
0.74%
New to Machine Learning? Start here.

Open new doors with Coursera Plus
Unlimited access to 7,000+ world-class courses, hands-on projects, and job-ready certificate programs - all included in your subscription
Advance your career with an online degree
Earn a degree from world-class universities - 100% online
Join over 3,400 global companies that choose Coursera for Business
Upskill your employees to excel in the digital economy
Frequently asked questions
Organizations of all types and sizes have business processes that generate massive volumes of data. Every moment, all sorts of information gets created by computers, the internet, phones, texts, streaming video, photographs, sensors, and much more. In the global digital landscape, data is increasingly imprecise, chaotic, and unstructured. As the speed and variety of data increases exponentially, organizations are struggling to keep pace.
Data science and advanced data analytics are part of a field of study that uses raw data to create new ways of modeling and understanding the unknown. To gain insights, businesses rely on data professionals to acquire, organize, and interpret data, which helps inform internal projects and processes. Data scientists and advanced data analysts rely on a combination of critical skills, including statistics, scientific methods, data analysis, and artificial intelligence.
A data professional is a term used to describe any individual who works with data and/or has data skills. At a minimum, a data professional is capable of exploring, cleaning, selecting, analyzing, and visualizing data. They may also be comfortable with writing code and have some familiarity with the techniques used by statisticians and machine learning engineers, including building models, developing algorithmic thinking, and building machine learning models.
Data professionals are responsible for collecting, analyzing, and interpreting large amounts of data within a variety of different organizations. The role of a data professional is defined differently across companies. Generally speaking, data professionals possess technical and strategic capabilities that require more advanced analytical skills such as data manipulation, experimental design, predictive modeling, and machine learning. They perform a variety of tasks related to gathering, structuring, interpreting, monitoring, and reporting data in accessible formats, enabling stakeholders to understand and use data effectively. Ultimately, the work of data professionals helps organizations make informed, ethical decisions.
Large volumes of data — and the technology needed to manage and analyze it — are becoming increasingly accessible. Because of this, there has been a surge in career opportunities for people who can tell stories using data, such as senior data analysts and data scientists. These professionals collect, analyze, and interpret large amounts of data within a variety of different organizations. Their responsibilities require advanced analytical skills such as data manipulation, experimental design, predictive modeling, and machine learning.