This course introduces data analysis methods used in systems biology, bioinformatics, and systems pharmacology research. The course covers methods to process raw data from genome-wide mRNA expression studies (microarrays and RNA-seq) including data normalization, clustering, dimensionality reduction, differential expression, enrichment analysis, and network construction. The course contains practical tutorials for using several bioinformatics tools and setting up data analysis pipelines, also covering the mathematics behind the methods applied by these tools and workflows. The course is mostly appropriate for beginning graduate students and advanced undergraduates majoring in fields such as biology, statistics, physics, chemistry, computer science, biomedical and electrical engineering. The course should be useful for wet- and dry-lab researchers who encounter large datasets in their own research. The course presents software tools developed by the Ma’ayan Laboratory (http://labs.icahn.mssm.edu/maayanlab/) from the Icahn School of Medicine at Mount Sinai in New York City, but also other freely available data analysis and visualization tools. The overarching goal of the course is to enable students to utilize the methods presented in this course for analyzing their own data for their own projects. For those students that do not work in the field, the course introduces research challenges faced in the fields of computational systems biology and systems pharmacology.

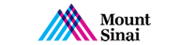
Network Analysis in Systems Biology
This course is part of Systems Biology and Biotechnology Specialization
Taught in English
Some content may not be translated

Instructor: Avi Ma’ayan, PhD
21,047 already enrolled
Included with
Course
(195 reviews)
92%
Details to know

Add to your LinkedIn profile
38 quizzes
Course
(195 reviews)
92%
See how employees at top companies are mastering in-demand skills
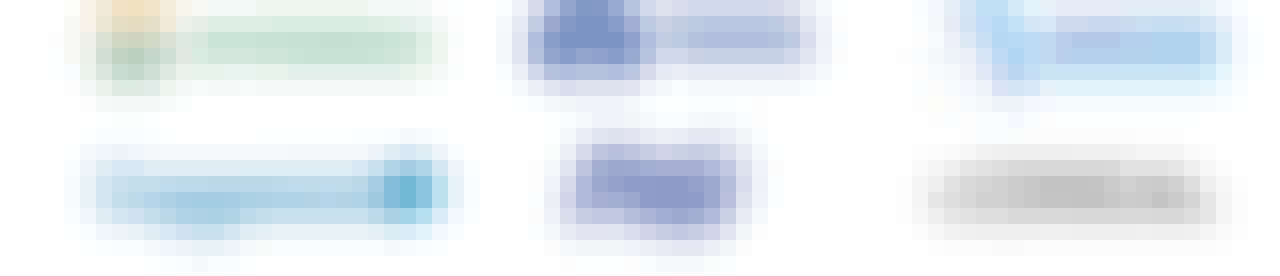
Build your subject-matter expertise
- Learn new concepts from industry experts
- Gain a foundational understanding of a subject or tool
- Develop job-relevant skills with hands-on projects
- Earn a shareable career certificate
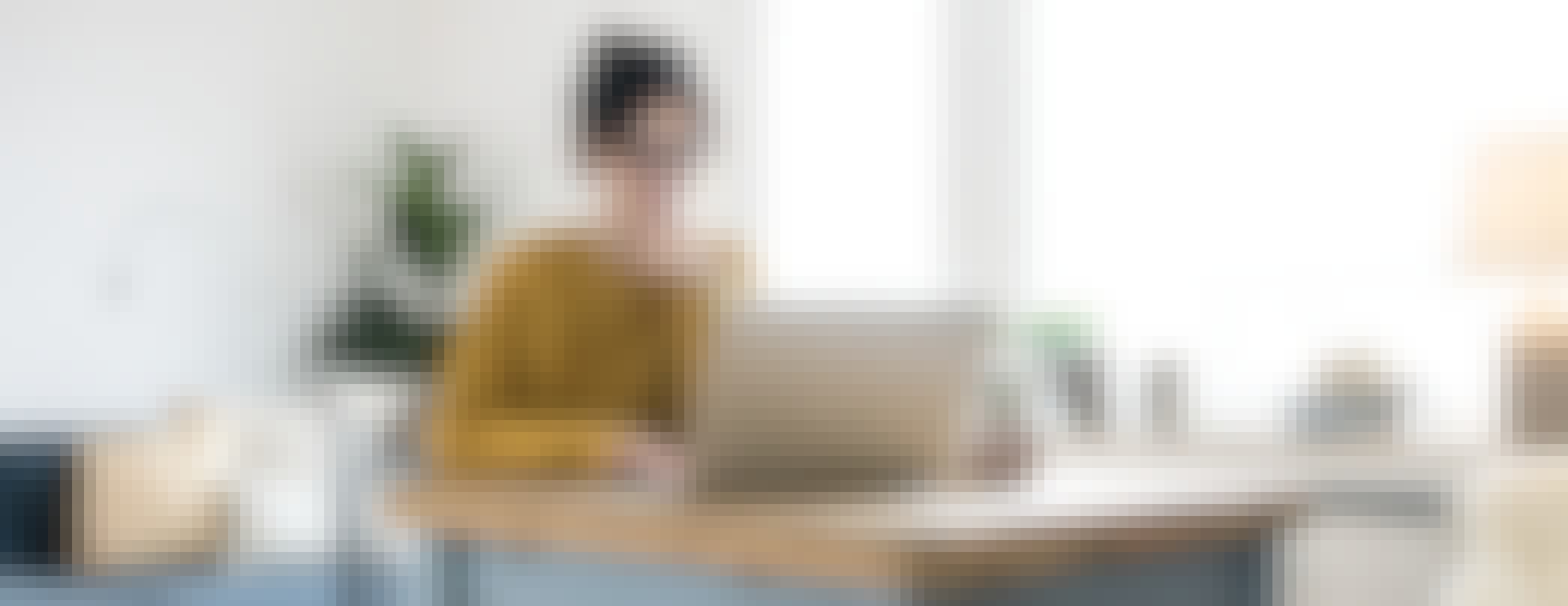
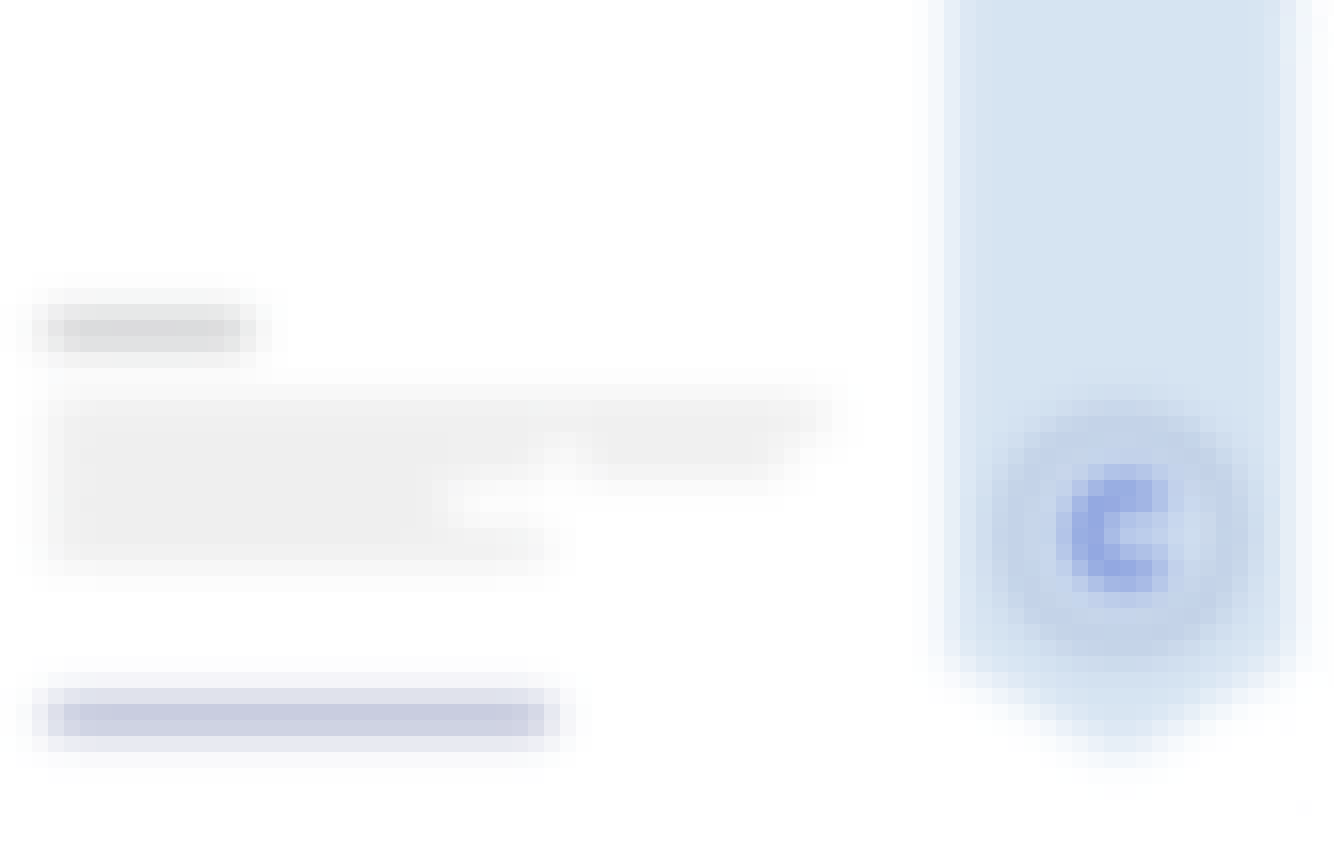
Earn a career certificate
Add this credential to your LinkedIn profile, resume, or CV
Share it on social media and in your performance review
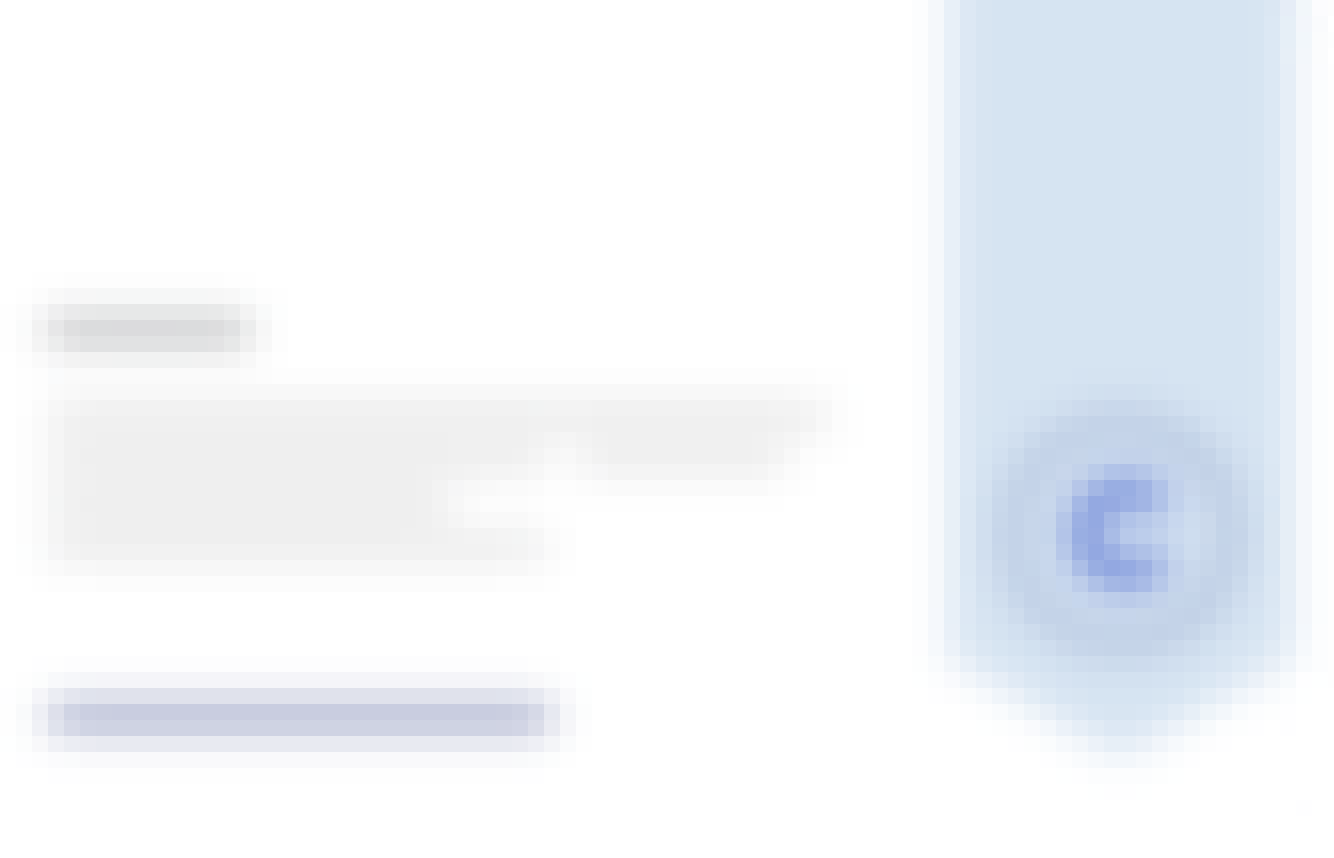
There are 10 modules in this course
The 'Introduction to Complex Systems' module discusses complex systems and leads to the idea that a cell can be considered a complex system or a complex agent living in a complex environment just like us. The 'Introduction to Biology for Engineers' module provides an introduction to some central topics in cell and molecular biology for those who do not have the background in the field. This is not a comprehensive coverage of cell and molecular biology. The goal is to provide an entry point to motivate those who are interested in this field, coming from other disciplines, to begin studying biology.
What's included
3 videos4 readings3 quizzes
In the 'Topological and Network Evolution Models' module, we provide several lectures about a historical perspective of network analysis in systems biology. The focus is on in-silico network evolution models. These are simple computational models that, based of few rules, can create networks that have a similar topology to the molecular networks observed in biological systems.
What's included
4 videos4 quizzes
The 'Types of Biological Networks' module is about the various types of networks that are typically constructed and analyzed in systems biology and systems pharmacology. This lecture ends with the idea of functional association networks (FANs). Following this lecture are lectures that discuss how to construct FANs and how to use these networks for analyzing gene lists.
What's included
4 videos4 quizzes
This set of lectures in the 'Data Processing and Identifying Differentially Expressed Genes' module first discusses data normalization methods, and then several lectures are devoted to explaining the problem of identifying differentially expressed genes with the focus on understanding the inner workings of a new method developed by the Ma'ayan Laboratory called the Characteristic Direction.
What's included
5 videos2 quizzes
In the 'Gene Set Enrichment and Network Analyses' module the emphasis is on tools developed by the Ma'ayan Laboratory to analyze gene sets. Several tools will be discussed including: Enrichr, GEO2Enrichr, Expression2Kinases and DrugPairSeeker. In addition, one lecture will be devoted to a method we call enrichment vector clustering we developed, and two lectures will describe the popular gene set enrichment analysis (GSEA) method and an improved method we developed called principal angle enrichment analysis (PAEA).
What's included
9 videos1 reading8 quizzes
A set of lectures in the 'Deep Sequencing Data Processing and Analysis' module will cover the basic steps and popular pipelines to analyze RNA-seq and ChIP-seq data going from the raw data to gene lists to figures. These lectures also cover UNIX/Linux commands and some programming elements of R, a popular freely available statistical software. Note that since these lectures were developed and recorded during the Fall of 2013, it is possible that there are better tools that should be used now since the field is rapidly advancing.
What's included
7 videos7 quizzes
This module is devoted to various method of clustering: principal component analysis, self-organizing maps, network-based clustering and hierarchical clustering. The theory behind these methods of analysis are covered in detail, and this is followed by some practical demonstration of the methods for applications using R and MATLAB.
What's included
6 videos1 reading6 quizzes
The lectures in the 'Resources for Data Integration' module are about the various types of networks that are typically constructed and analyzed in systems biology and systems pharmacology. These lectures start with the idea of functional association networks (FANs). Following this lecture are several lectures that discuss how to construct FANs from various resources and how to use these networks for analyzing gene lists as well as to construct a puzzle that can be used to connect genomic data with phenotypic data.
What's included
5 videos2 quizzes
The final set of lectures presents the idea of crowdsourcing. MOOCs provide the opportunity to work together on projects that are difficult to complete alone (microtasks) or compete for implementing the best algorithms to solve hard problems (megatasks). You will have the opportunity to participate in various crowdsourcing projects: microtasks and megatasks. These projects are designed specifically for this course.
What's included
2 videos1 quiz
The final exam consists of multiple choice questions from topics covered in all of modules of the course. Some of the questions may require you to perform some of the analysis methods you learned throughout the course on new datasets.
What's included
1 quiz
Instructor

Offered by
Recommended if you're interested in Basic Science
Icahn School of Medicine at Mount Sinai
Icahn School of Medicine at Mount Sinai
Icahn School of Medicine at Mount Sinai
Icahn School of Medicine at Mount Sinai
Why people choose Coursera for their career




Learner reviews
Showing 3 of 195
195 reviews
- 5 stars
63.77%
- 4 stars
24.48%
- 3 stars
8.67%
- 2 stars
0.51%
- 1 star
2.55%
New to Basic Science? Start here.

Open new doors with Coursera Plus
Unlimited access to 7,000+ world-class courses, hands-on projects, and job-ready certificate programs - all included in your subscription
Advance your career with an online degree
Earn a degree from world-class universities - 100% online
Join over 3,400 global companies that choose Coursera for Business
Upskill your employees to excel in the digital economy
Frequently asked questions
Access to lectures and assignments depends on your type of enrollment. If you take a course in audit mode, you will be able to see most course materials for free. To access graded assignments and to earn a Certificate, you will need to purchase the Certificate experience, during or after your audit. If you don't see the audit option:
The course may not offer an audit option. You can try a Free Trial instead, or apply for Financial Aid.
The course may offer 'Full Course, No Certificate' instead. This option lets you see all course materials, submit required assessments, and get a final grade. This also means that you will not be able to purchase a Certificate experience.
When you enroll in the course, you get access to all of the courses in the Specialization, and you earn a certificate when you complete the work. Your electronic Certificate will be added to your Accomplishments page - from there, you can print your Certificate or add it to your LinkedIn profile. If you only want to read and view the course content, you can audit the course for free.
If you subscribed, you get a 7-day free trial during which you can cancel at no penalty. After that, we don’t give refunds, but you can cancel your subscription at any time. See our full refund policy.