In this 2-hour long project, you will learn how to analyze a dataset for sentiment analysis. You will learn how to read in a PyTorch BERT model, and adjust the architecture for multi-class classification. You will learn how to adjust an optimizer and scheduler for ideal training and performance. In fine-tuning this model, you will learn how to design a train and evaluate loop to monitor model performance as it trains, including saving and loading models. Finally, you will build a Sentiment Analysis model that leverages BERT's large-scale language knowledge.

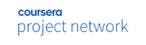
Sentiment Analysis with Deep Learning using BERT
Taught in English

Instructor: Ari Anastassiou
14,863 already enrolled
Included with
Guided Project
Recommended experience
(392 reviews)
What you'll learn
Preprocess and clean data for BERT Classification
Load in pretrained BERT with custom output layer
Train and evaluate finetuned BERT architecture on your own problem statement
Skills you'll practice
Details to know

Add to your LinkedIn profile
Guided Project
Recommended experience
(392 reviews)
See how employees at top companies are mastering in-demand skills
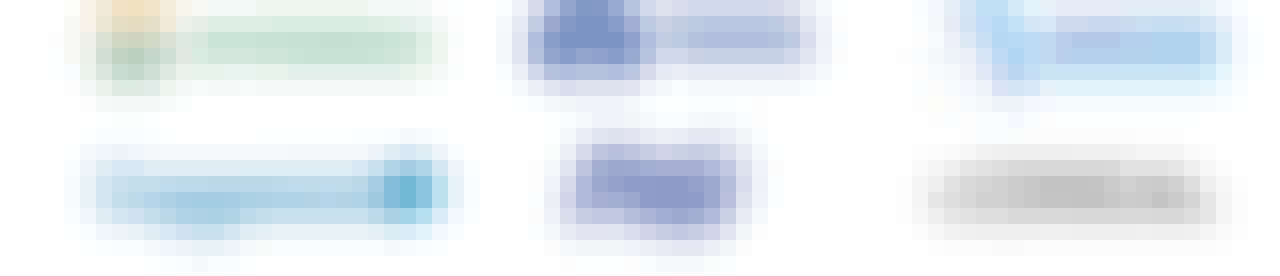
Learn, practice, and apply job-ready skills in less than 2 hours
- Receive training from industry experts
- Gain hands-on experience solving real-world job tasks
- Build confidence using the latest tools and technologies
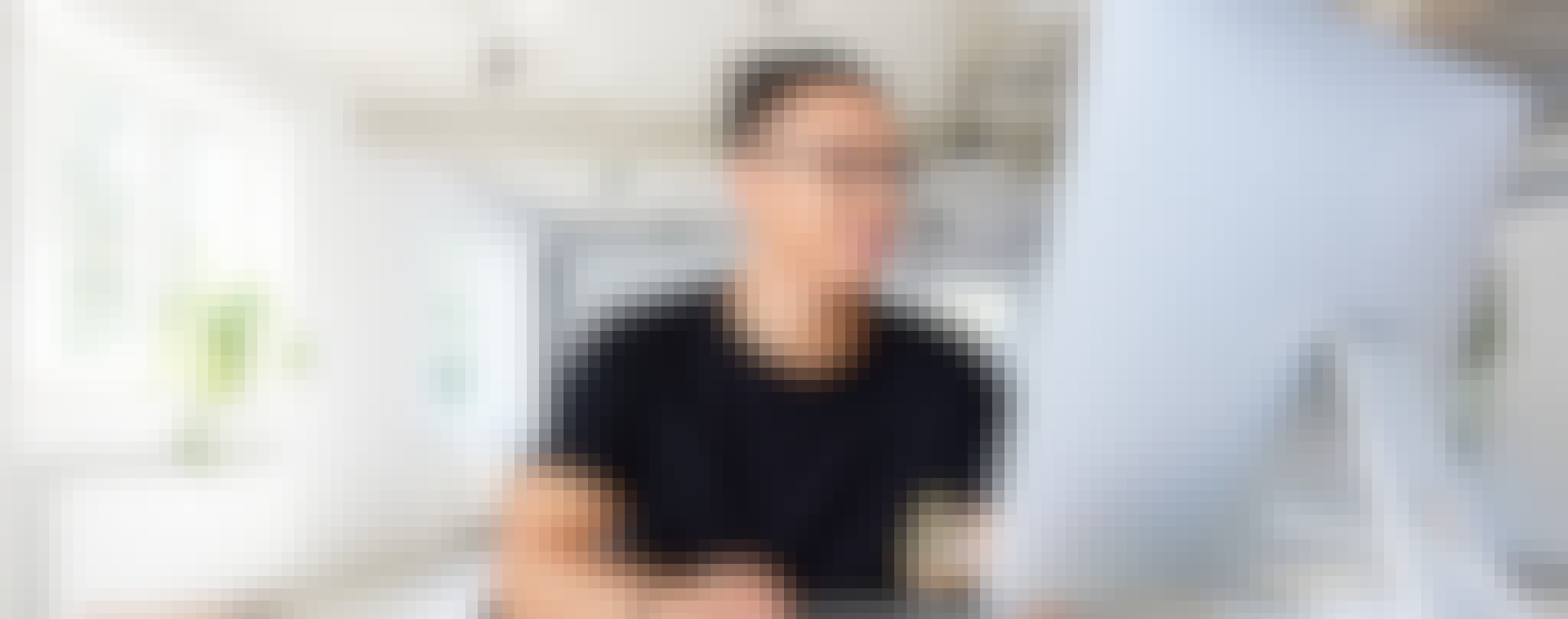
About this Guided Project
Learn step-by-step
In a video that plays in a split-screen with your work area, your instructor will walk you through these steps:
Introduction to BERT and the problem at hand
Exploratory Data Analysis and Preprocessing
Training/Validation Split
Loading Tokenizer and Encoding our Data
Setting up BERT Pretrained Model
Creating Data Loaders
Setting Up Optimizer and Scheduler
Defining our Performance Metrics
Creating our Training Loop
Loading and Evaluating our Model
Recommended experience
Intermediate Python users with some exposure to NumPy, Pandas, and PyTorch.
2 project images
Instructor

Offered by
How you'll learn
Skill-based, hands-on learning
Practice new skills by completing job-related tasks.
Expert guidance
Follow along with pre-recorded videos from experts using a unique side-by-side interface.
No downloads or installation required
Access the tools and resources you need in a pre-configured cloud workspace.
Available only on desktop
This Guided Project is designed for laptops or desktop computers with a reliable Internet connection, not mobile devices.
Why people choose Coursera for their career




Learner reviews
Showing 3 of 392
392 reviews
- 5 stars
62.34%
- 4 stars
25.95%
- 3 stars
7.88%
- 2 stars
2.03%
- 1 star
1.78%
New to Machine Learning? Start here.

Open new doors with Coursera Plus
Unlimited access to 7,000+ world-class courses, hands-on projects, and job-ready certificate programs - all included in your subscription
Advance your career with an online degree
Earn a degree from world-class universities - 100% online
Join over 3,400 global companies that choose Coursera for Business
Upskill your employees to excel in the digital economy
Frequently asked questions
By purchasing a Guided Project, you'll get everything you need to complete the Guided Project including access to a cloud desktop workspace through your web browser that contains the files and software you need to get started, plus step-by-step video instruction from a subject matter expert.
Because your workspace contains a cloud desktop that is sized for a laptop or desktop computer, Guided Projects are not available on your mobile device.
Guided Project instructors are subject matter experts who have experience in the skill, tool or domain of their project and are passionate about sharing their knowledge to impact millions of learners around the world.