Do you have data and wonder what it can tell you? Do you need a deeper understanding of the core ways in which machine learning can improve your business? Do you want to be able to converse with specialists about anything from regression and classification to deep learning and recommender systems?

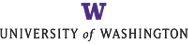
Machine Learning Foundations: A Case Study Approach
This course is part of Machine Learning Specialization
Taught in English
Some content may not be translated


Instructors: Emily Fox
389,080 already enrolled
Included with
Course
(13,376 reviews)
93%
Skills you'll gain
Details to know

Add to your LinkedIn profile
11 quizzes
Course
(13,376 reviews)
93%
See how employees at top companies are mastering in-demand skills
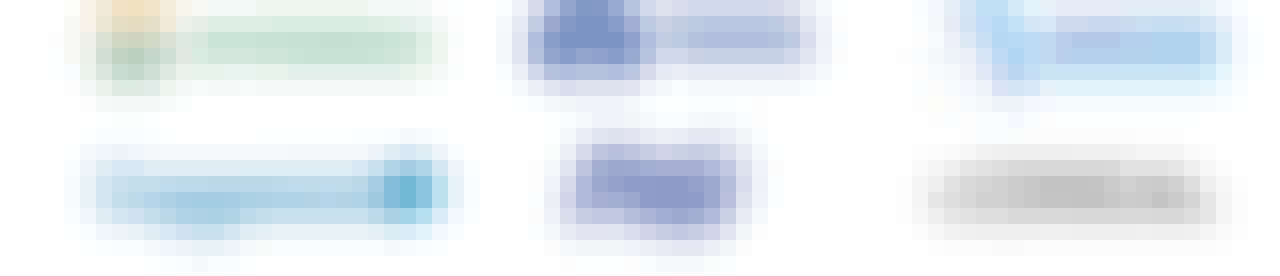
Build your subject-matter expertise
- Learn new concepts from industry experts
- Gain a foundational understanding of a subject or tool
- Develop job-relevant skills with hands-on projects
- Earn a shareable career certificate
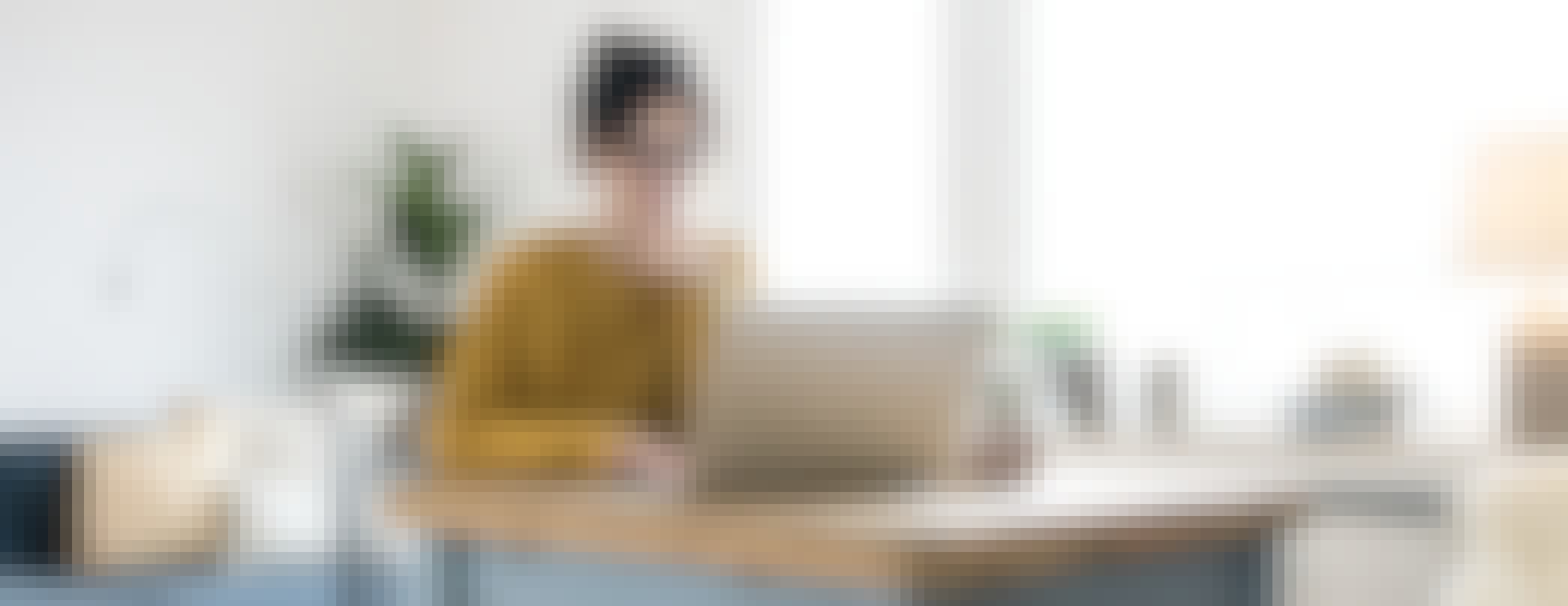
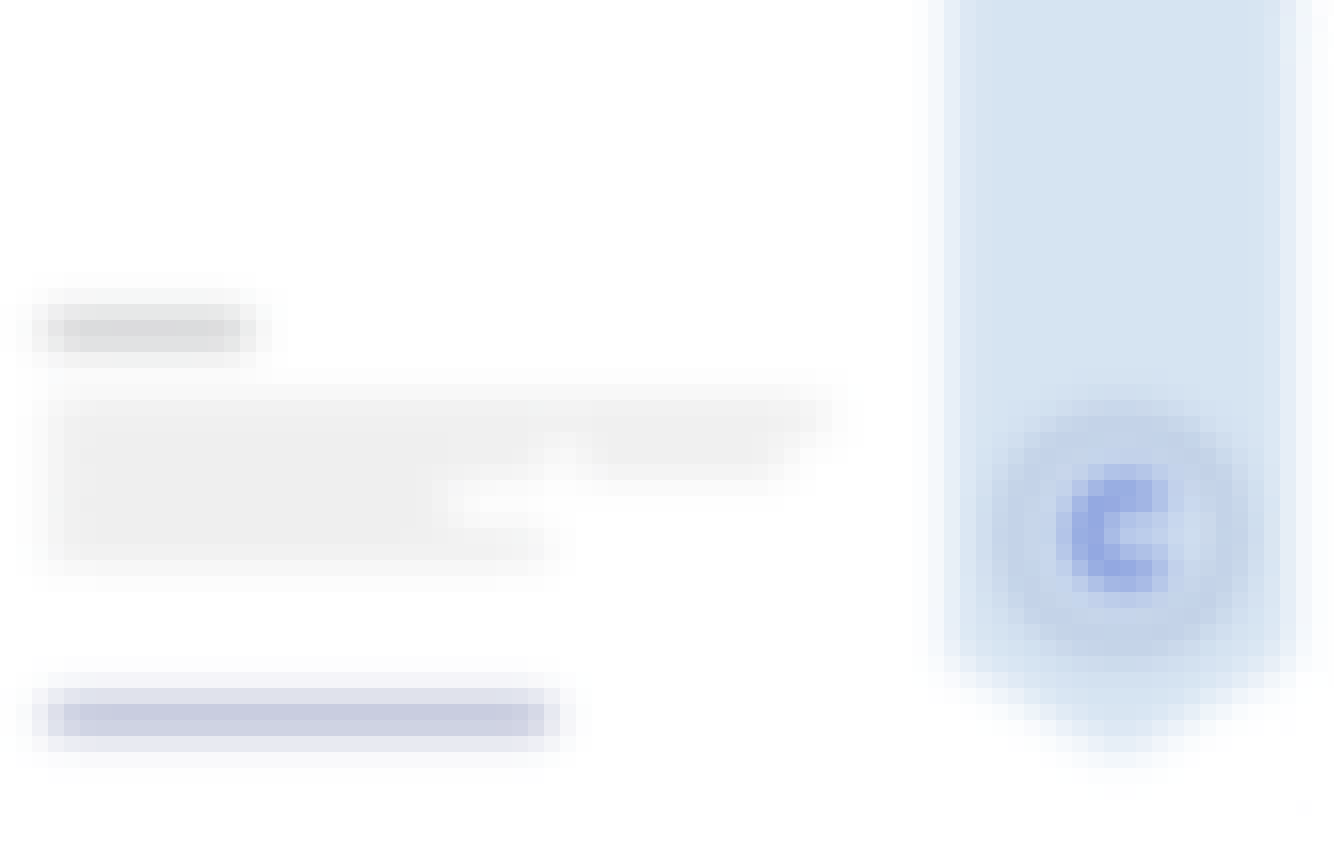
Earn a career certificate
Add this credential to your LinkedIn profile, resume, or CV
Share it on social media and in your performance review
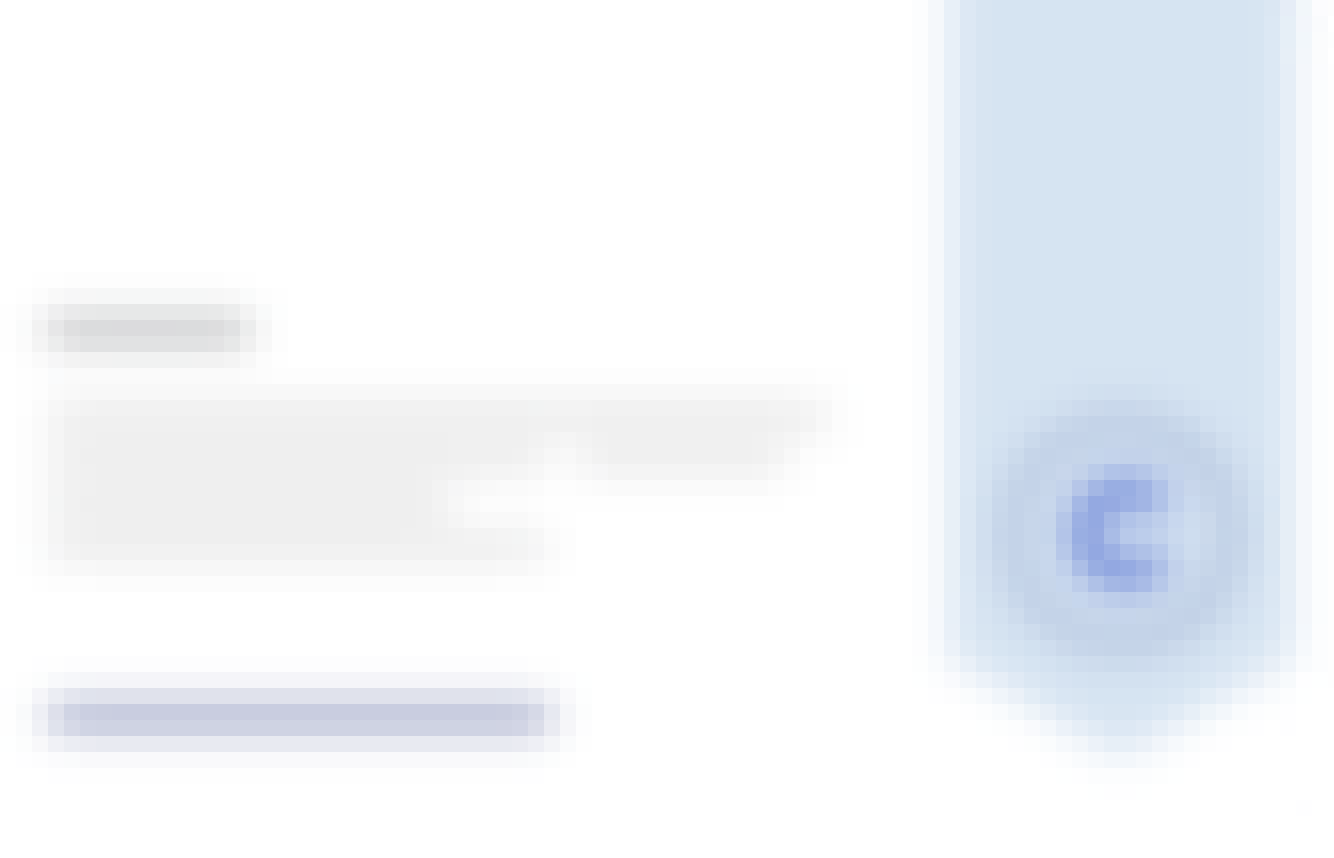
There are 7 modules in this course
Machine learning is everywhere, but is often operating behind the scenes. <p>This introduction to the specialization provides you with insights into the power of machine learning, and the multitude of intelligent applications you personally will be able to develop and deploy upon completion.</p>We also discuss who we are, how we got here, and our view of the future of intelligent applications.
What's included
18 videos9 readings1 quiz
This week you will build your first intelligent application that makes predictions from data.<p>We will explore this idea within the context of our first case study, predicting house prices, where you will create models that predict a continuous value (price) from input features (square footage, number of bedrooms and bathrooms,...). <p>This is just one of the many places where regression can be applied.Other applications range from predicting health outcomes in medicine, stock prices in finance, and power usage in high-performance computing, to analyzing which regulators are important for gene expression.</p>You will also examine how to analyze the performance of your predictive model and implement regression in practice using a Jupyter notebook.
What's included
19 videos3 readings2 quizzes
How do you guess whether a person felt positively or negatively about an experience, just from a short review they wrote?<p>In our second case study, analyzing sentiment, you will create models that predict a class (positive/negative sentiment) from input features (text of the reviews, user profile information,...).This task is an example of classification, one of the most widely used areas of machine learning, with a broad array of applications, including ad targeting, spam detection, medical diagnosis and image classification.</p>You will analyze the accuracy of your classifier, implement an actual classifier in a Jupyter notebook, and take a first stab at a core piece of the intelligent application you will build and deploy in your capstone.
What's included
19 videos3 readings2 quizzes
A reader is interested in a specific news article and you want to find a similar articles to recommend. What is the right notion of similarity? How do I automatically search over documents to find the one that is most similar? How do I quantitatively represent the documents in the first place?<p>In this third case study, retrieving documents, you will examine various document representations and an algorithm to retrieve the most similar subset. You will also consider structured representations of the documents that automatically group articles by similarity (e.g., document topic).</p>You will actually build an intelligent document retrieval system for Wikipedia entries in an Jupyter notebook.
What's included
17 videos3 readings2 quizzes
Ever wonder how Amazon forms its personalized product recommendations? How Netflix suggests movies to watch? How Pandora selects the next song to stream? How Facebook or LinkedIn finds people you might connect with? Underlying all of these technologies for personalized content is something called collaborative filtering. <p>You will learn how to build such a recommender system using a variety of techniques, and explore their tradeoffs.</p> One method we examine is matrix factorization, which learns features of users and products to form recommendations. In a Jupyter notebook, you will use these techniques to build a real song recommender system.
What's included
19 videos3 readings2 quizzes
You’ve probably heard that Deep Learning is making news across the world as one of the most promising techniques in machine learning. Every industry is dedicating resources to unlock the deep learning potential, including for tasks such as image tagging, object recognition, speech recognition, and text analysis.<p>In our final case study, searching for images, you will learn how layers of neural networks provide very descriptive (non-linear) features that provide impressive performance in image classification and retrieval tasks. You will then construct deep features, a transfer learning technique that allows you to use deep learning very easily, even when you have little data to train the model.</p>Using iPhython notebooks, you will build an image classifier and an intelligent image retrieval system with deep learning.
What's included
18 videos4 readings2 quizzes
In the conclusion of the course, we will describe the final stage in turning our machine learning tools into a service: deployment.<p>We will also discuss some open challenges that the field of machine learning still faces, and where we think machine learning is heading. We conclude with an overview of what's in store for you in the rest of the specialization, and the amazing intelligent applications that are ahead for us as we evolve machine learning.
What's included
7 videos1 reading
Instructors


Offered by
Recommended if you're interested in Machine Learning
University of Washington
University of Washington
University of Washington
University of Washington
Why people choose Coursera for their career




Learner reviews
Showing 3 of 13376
13,376 reviews
- 5 stars
72.37%
- 4 stars
20.89%
- 3 stars
3.72%
- 2 stars
1.11%
- 1 star
1.89%
New to Machine Learning? Start here.

Open new doors with Coursera Plus
Unlimited access to 7,000+ world-class courses, hands-on projects, and job-ready certificate programs - all included in your subscription
Advance your career with an online degree
Earn a degree from world-class universities - 100% online
Join over 3,400 global companies that choose Coursera for Business
Upskill your employees to excel in the digital economy
Frequently asked questions
Access to lectures and assignments depends on your type of enrollment. If you take a course in audit mode, you will be able to see most course materials for free. To access graded assignments and to earn a Certificate, you will need to purchase the Certificate experience, during or after your audit. If you don't see the audit option:
The course may not offer an audit option. You can try a Free Trial instead, or apply for Financial Aid.
The course may offer 'Full Course, No Certificate' instead. This option lets you see all course materials, submit required assessments, and get a final grade. This also means that you will not be able to purchase a Certificate experience.
When you enroll in the course, you get access to all of the courses in the Specialization, and you earn a certificate when you complete the work. Your electronic Certificate will be added to your Accomplishments page - from there, you can print your Certificate or add it to your LinkedIn profile. If you only want to read and view the course content, you can audit the course for free.
If you subscribed, you get a 7-day free trial during which you can cancel at no penalty. After that, we don’t give refunds, but you can cancel your subscription at any time. See our full refund policy.